Will Natural Language Processing (NLP) Transform Warehouse Operations?
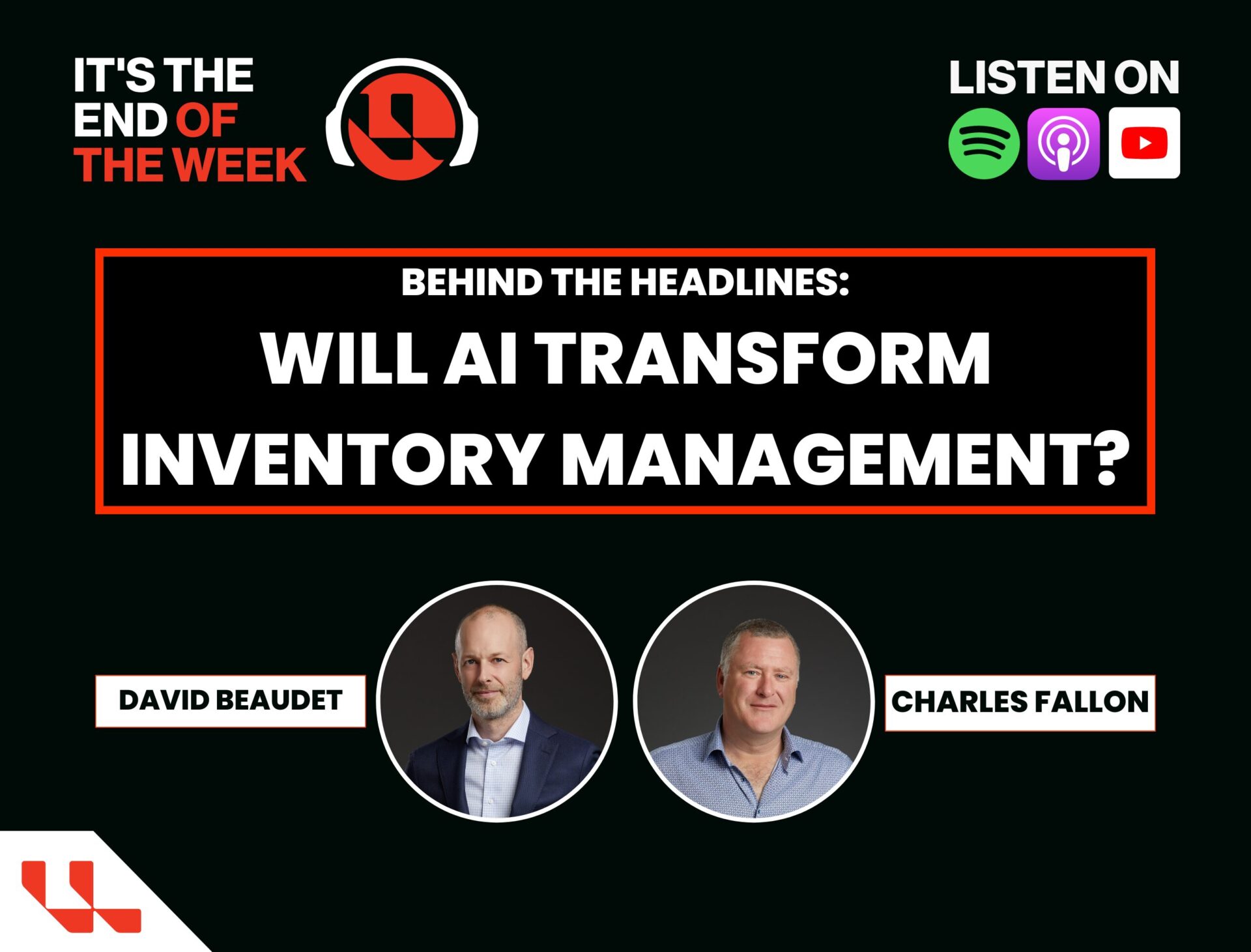
Introduction
In the past few years, artificial intelligence (AI) has promised to reshape industries worldwide, offering novel opportunities for innovation and efficiency. Within the realm of supply chain management, some people argue that AI-powered technologies like Natural Language Processing (NLP) are driving significant evolutions from traditional approaches to warehouse operations and inventory management. Through all the noise, it’s essential for decision makers to understand not just the potential benefits of AI and NLP, but also the challenges that accompany these advancements.
The Benefits
- Enhanced Operational Insights: NLP enables operators to extract valuable insights from unstructured data sources such as emails, customer feedback, and even verbal communications from frontline warehouse personnel. By converting this language into structured data, businesses gain deeper visibility into inventory dynamics and consumer trends for more effective warehouse operations.
- Real-Time Decisions: The insights gathered from previously unstructured sources can be leveraged in real-time, enabling decision-makers to make agile and proactive decisions based on the most up-to-date information from their warehouse and customers.
- Automation: With NLP technology, operators can automate traditionally manual tasks such as data entry and analysis which helps to streamline supply chain processes and reduce operational costs. This helps businesses to focus resources on strategic initiatives and value-added activities.
The Challenges
- Data Quality Challenges: NLP algorithms rely heavily on the quality of input data. Inaccuracies or inconsistencies in data sources can lead to errors in analysis and decision-making, undermining the effectiveness of NLP applications.
- Complexity and Scalability: Implementing NLP solutions in large-scale supply chain operations can be complex and resource-intensive. Businesses may face challenges related to data integration, system compatibility, and scalability, particularly when dealing with diverse data sources and formats.
- Ethical and Privacy Concerns: NLP raises ethical considerations related to data privacy and security. As businesses collect and analyze vast amounts of textual data, they must ensure compliance with data protection regulations and ethical guidelines to safeguard consumer privacy.
Conclusion
Implementing NLP into your warehouse operations could potentially provide huge benefits for a business, but operators need to be aware of key considerations regarding data, scalability, and privacy before making the jump. To learn more about this exciting topic, check out the accompanying podcast episode with LIDD founders Charles Fallon and David Beaudet discussing the emerging topic. If you have questions about how you can prepare your operations for the implementation AI and NLP, reach out directly to [email protected].
🔗 Check out the full episode:
Listen: Anywhere you get your podcasts.
Watch the full video below:
Related posts test
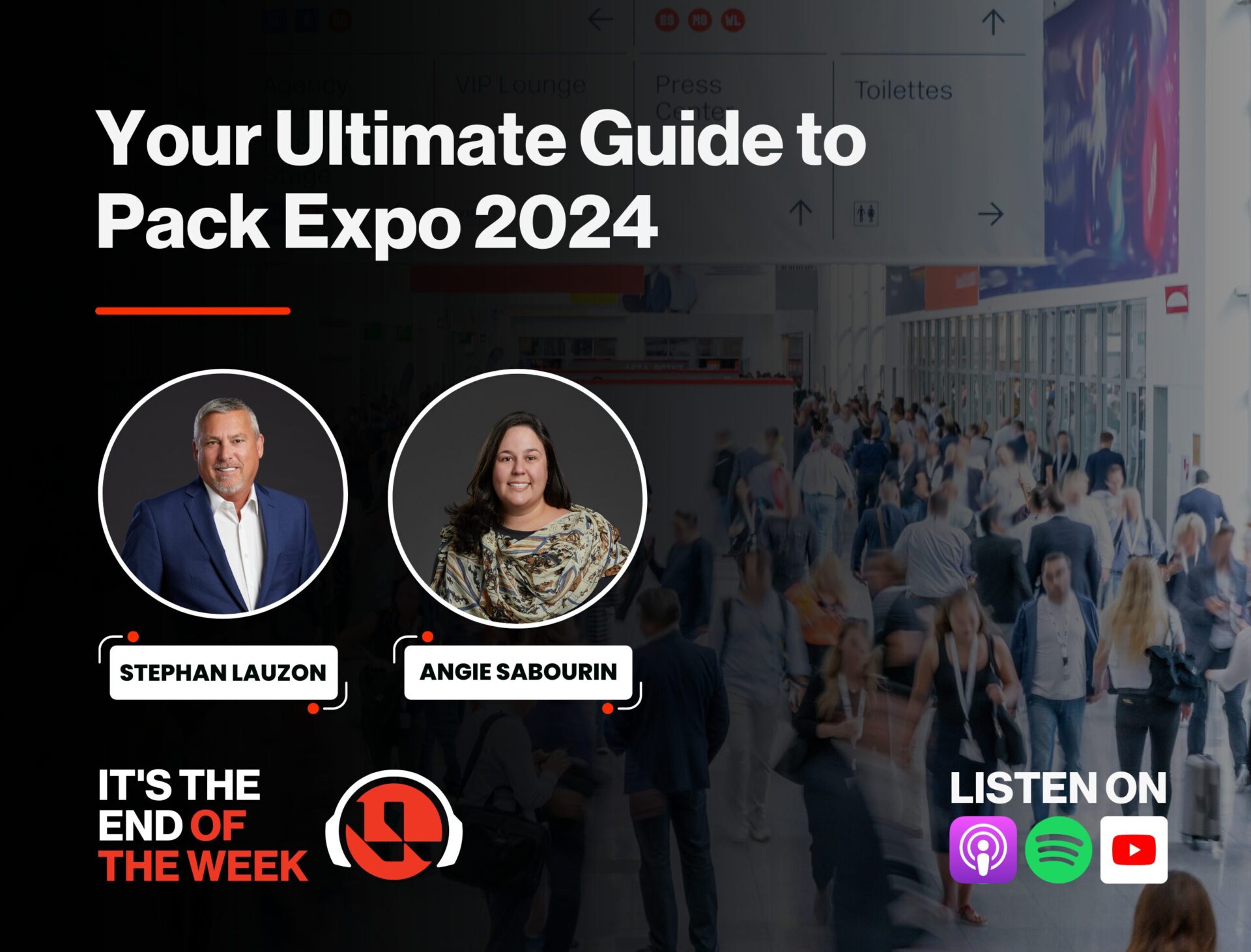
Your Ultimate Guide to Pack Expo 2024
Optimize your peak season planning with this comprehensive guide. Learn about staffing, automation,...
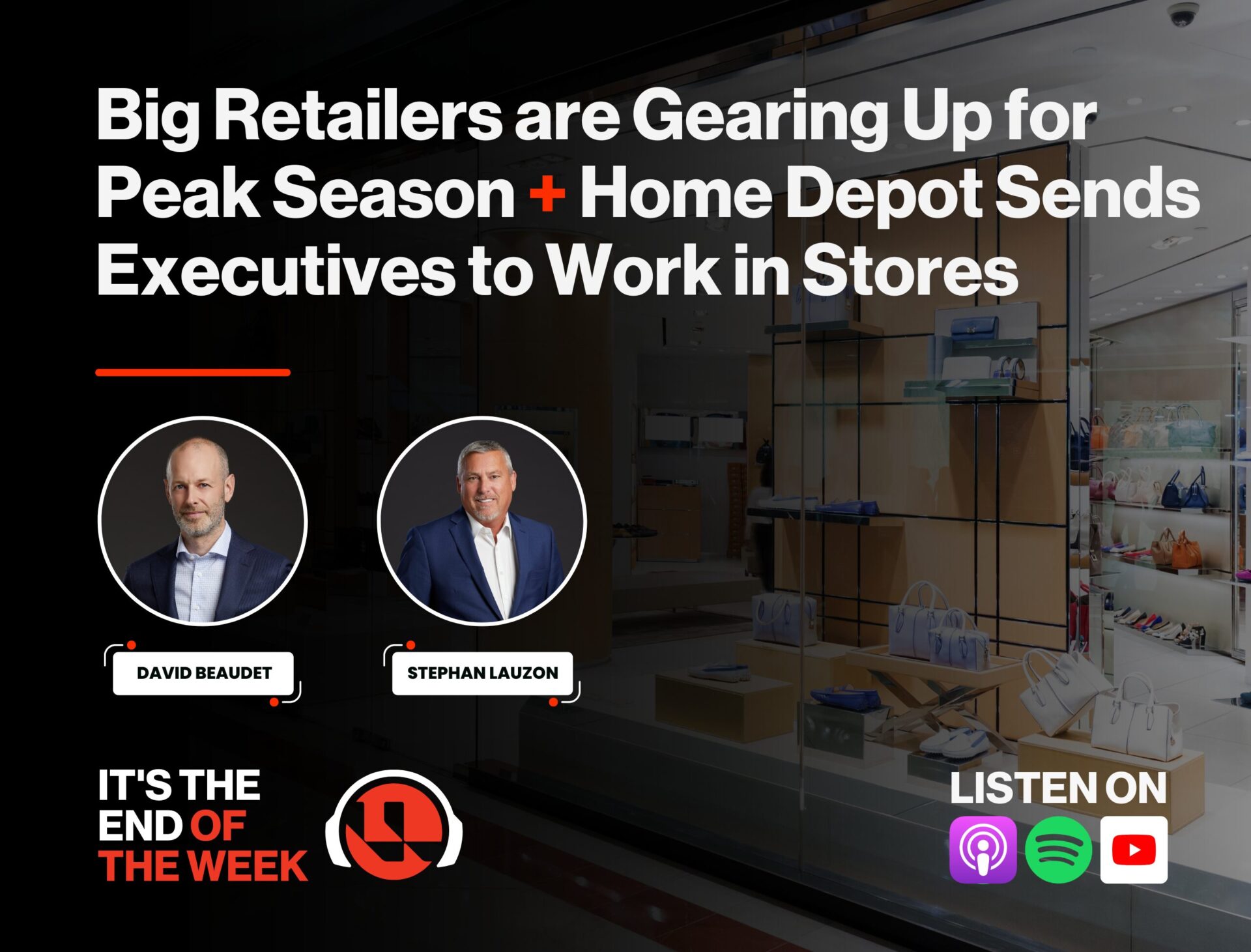
Peak Season Planning: Why You Need to Start Now
Optimize your peak season planning with this comprehensive guide. Learn about staffing, automation,...
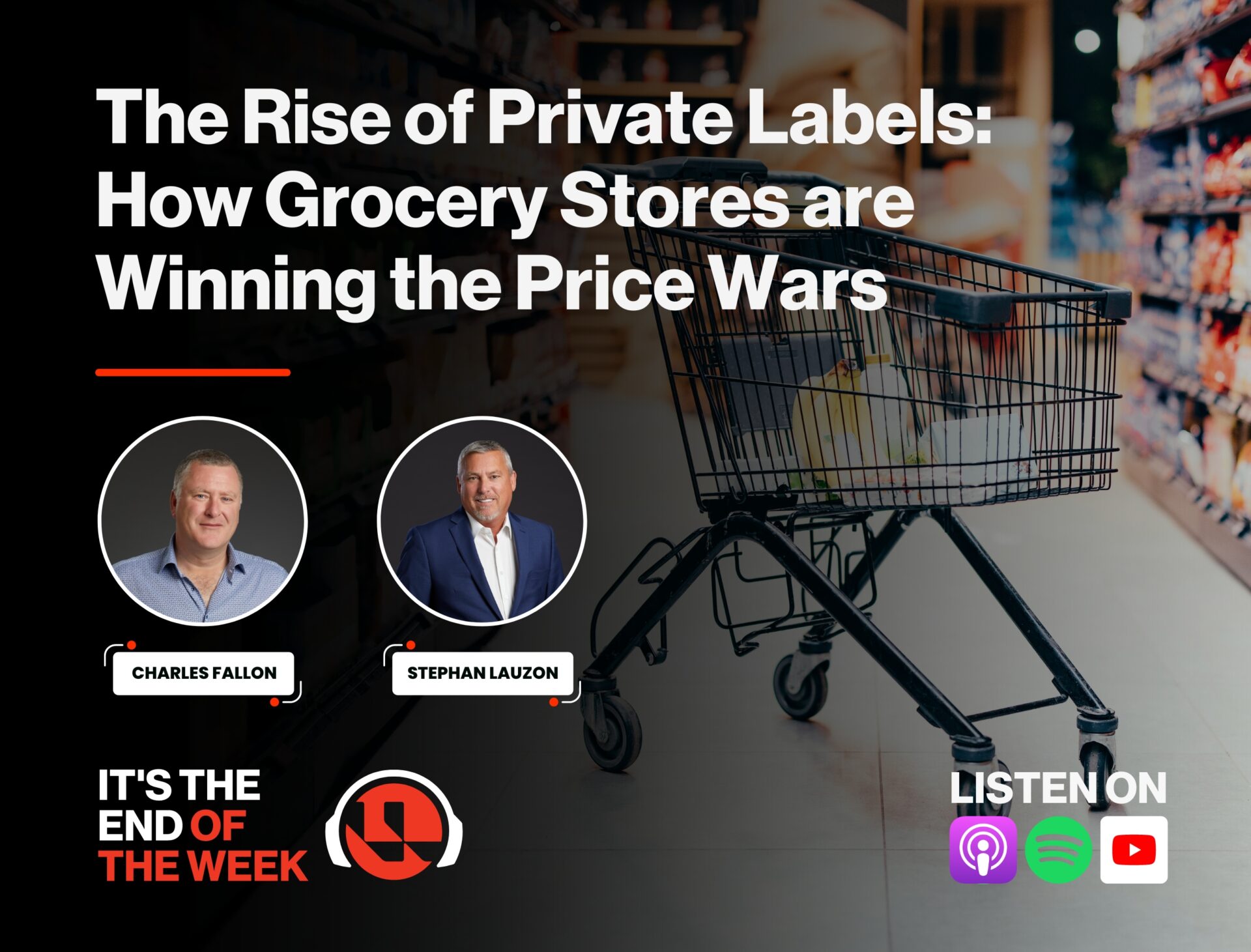
The Rise of Private Label: How Grocery Stores are Winning the Price Wars
Click to explore how GLP-1 drugs and the potential end of the de minimis rule could reshape the supp...
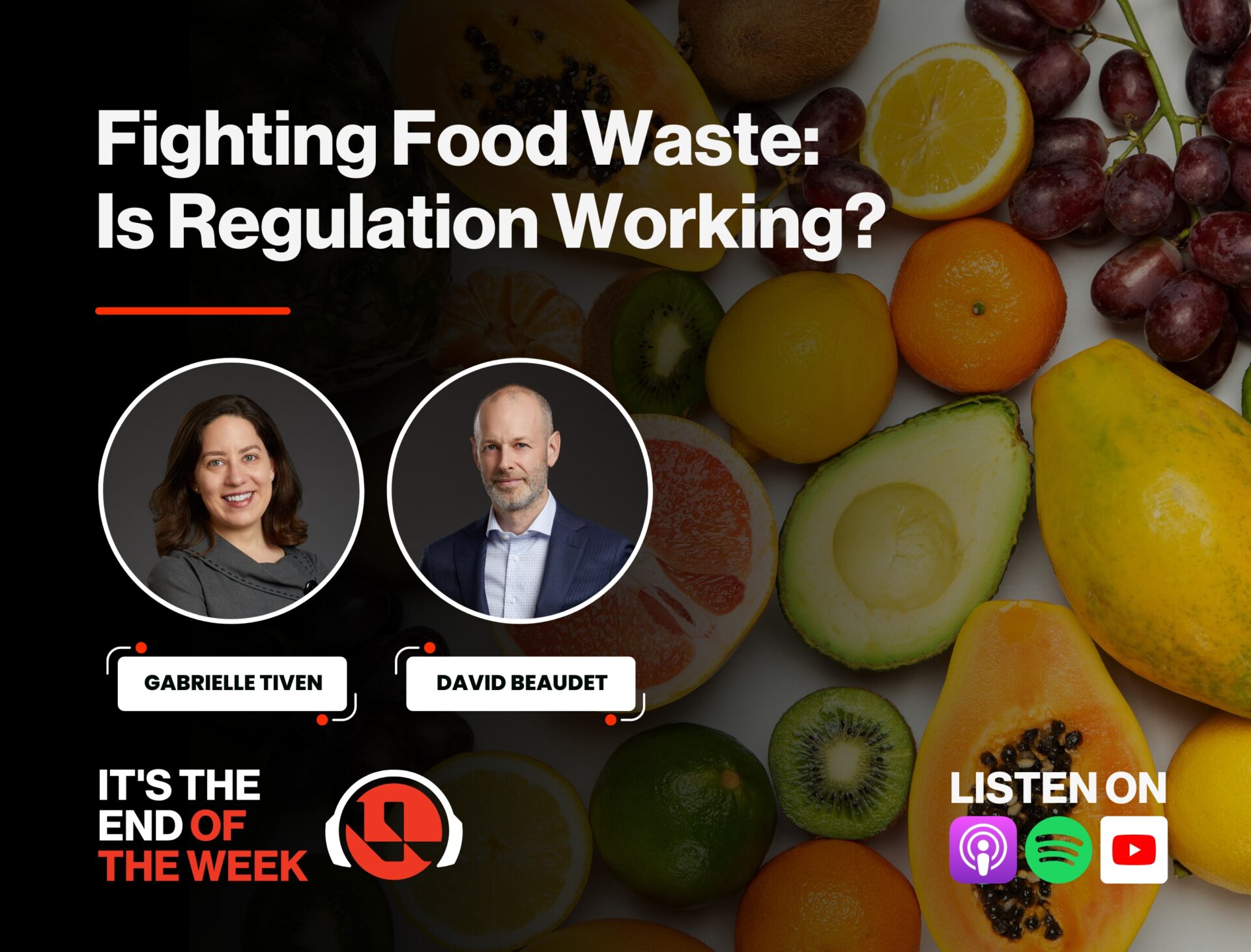
A Critical Look at Food Waste Regulation in Five States
Click to explore how GLP-1 drugs and the potential end of the de minimis rule could reshape the supp...
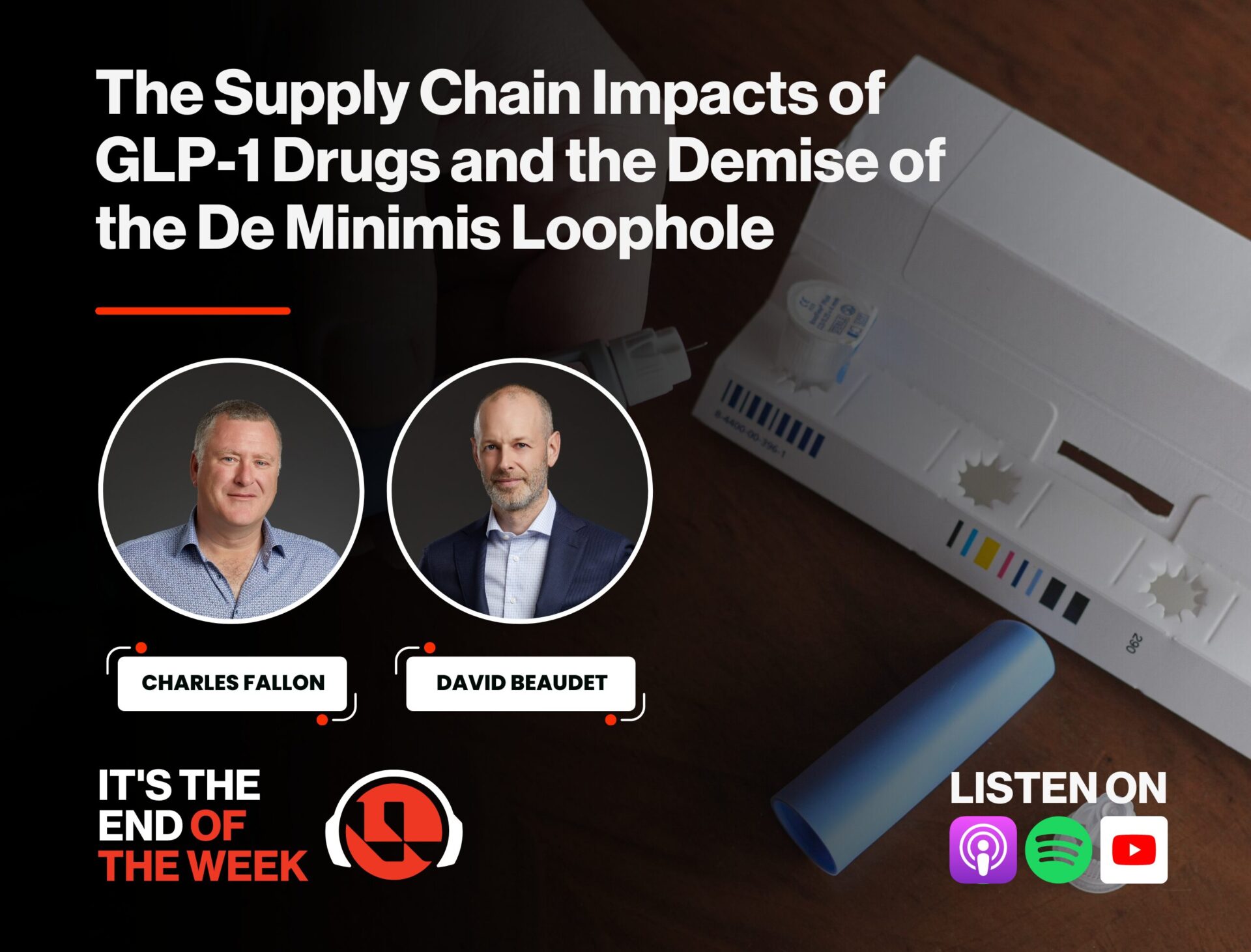
The Supply Chain Impacts of GLP-1 Drugs and the Demise of the De Minimis Loophole
Click to explore how GLP-1 drugs and the potential end of the de minimis rule could reshape the supp...
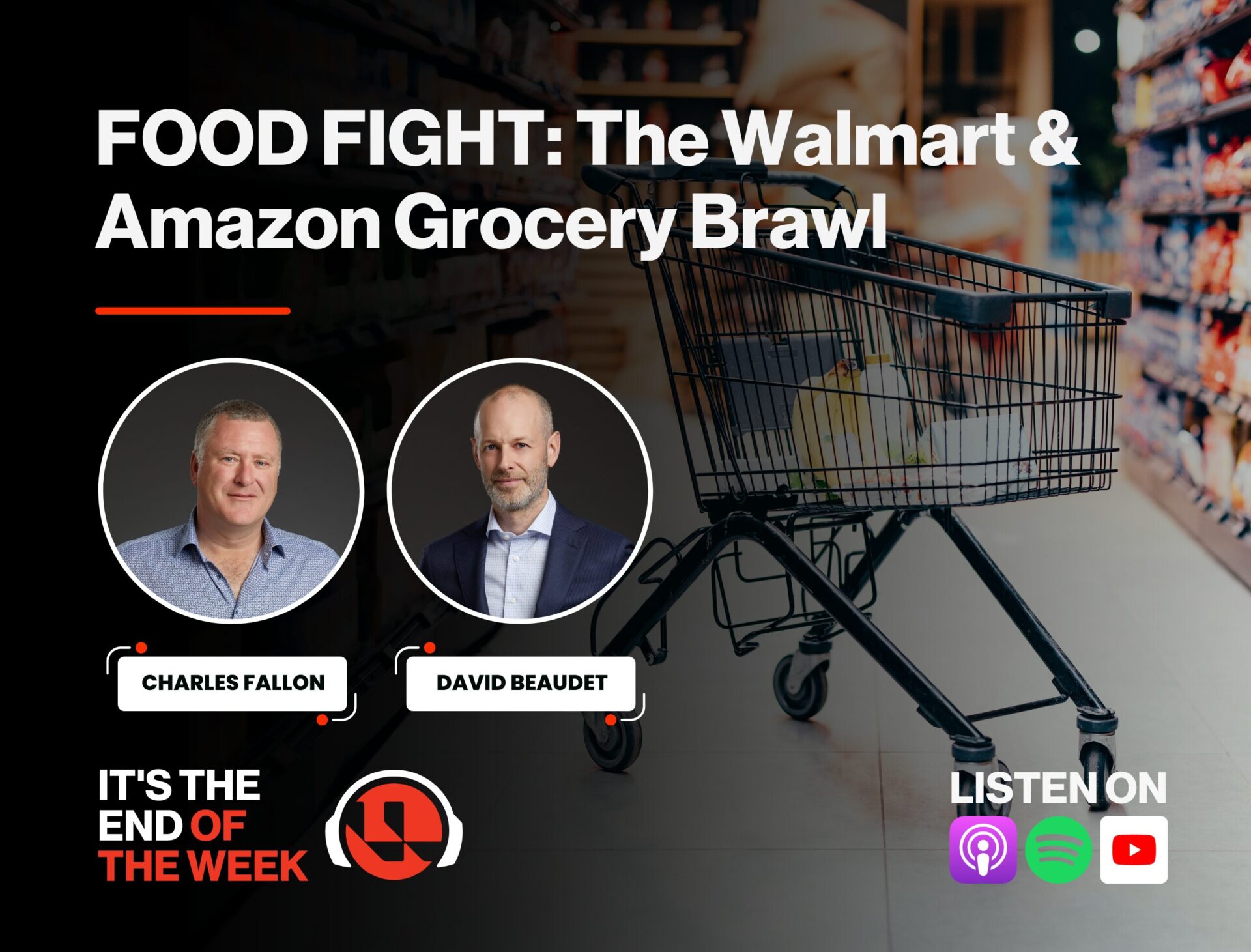
Food Fight: Inside the Walmart & Amazon Grocery Brawl
The grocery business food fight between Walmart and Amazon intensifies as Walmart leads in online gr...
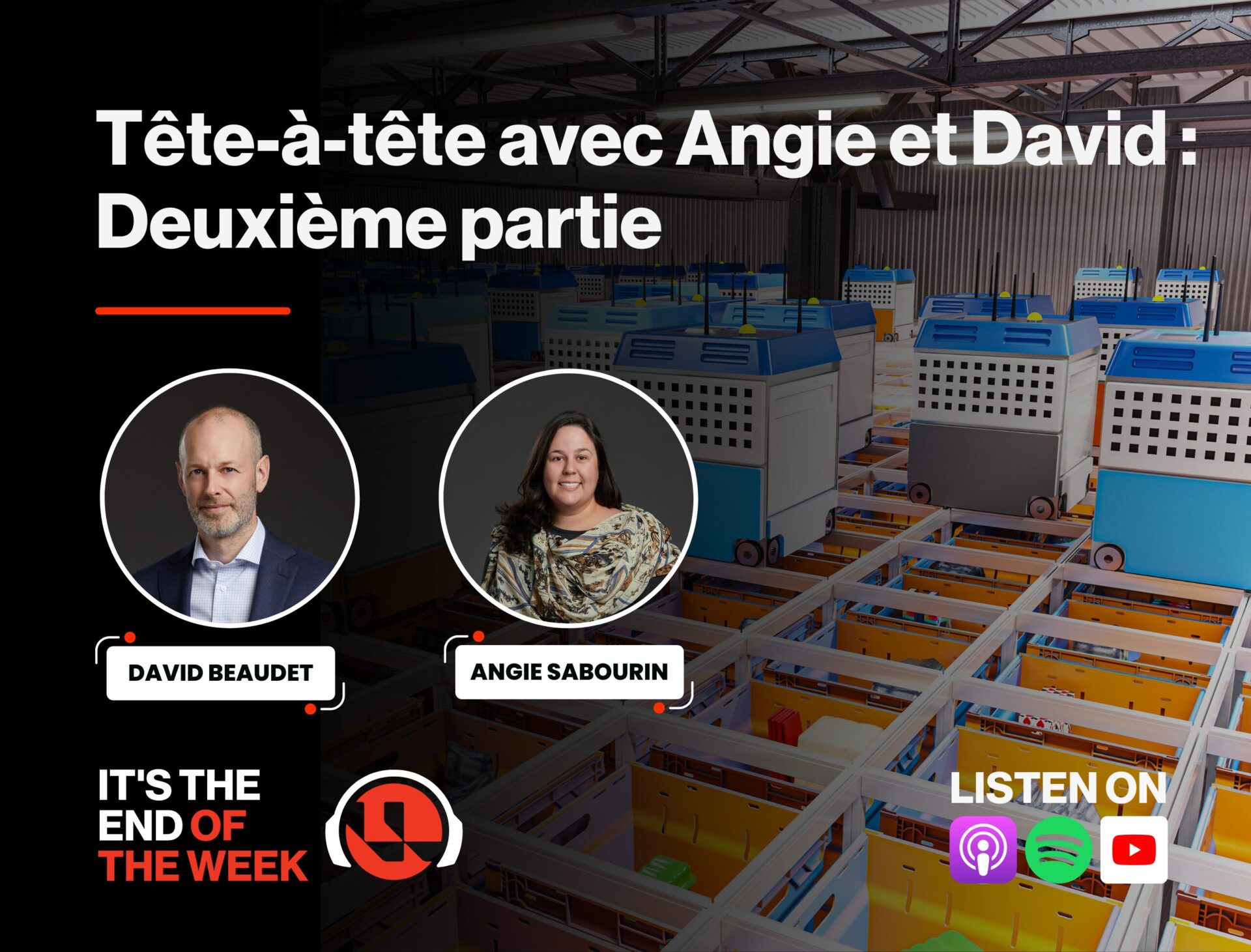
Tête à Tête with Angie and David: Discussing Warehouse Automation
Many Canadian businesses are looking to automate - but don't have the expertise to know where to get...
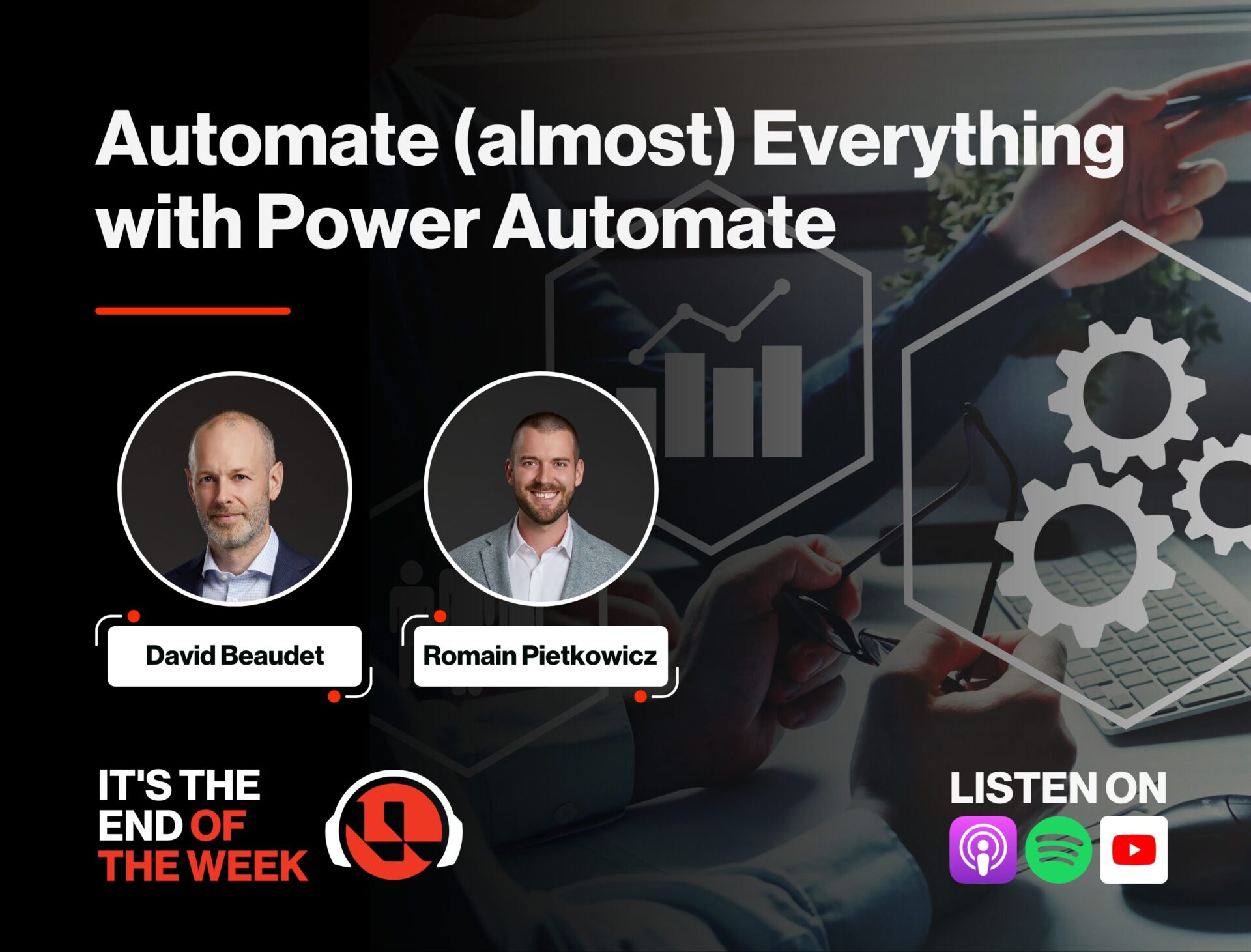
Automate (almost) Everything with Power Platform
Power Automate is a powerful platform designed to automate both internal and external facing process...
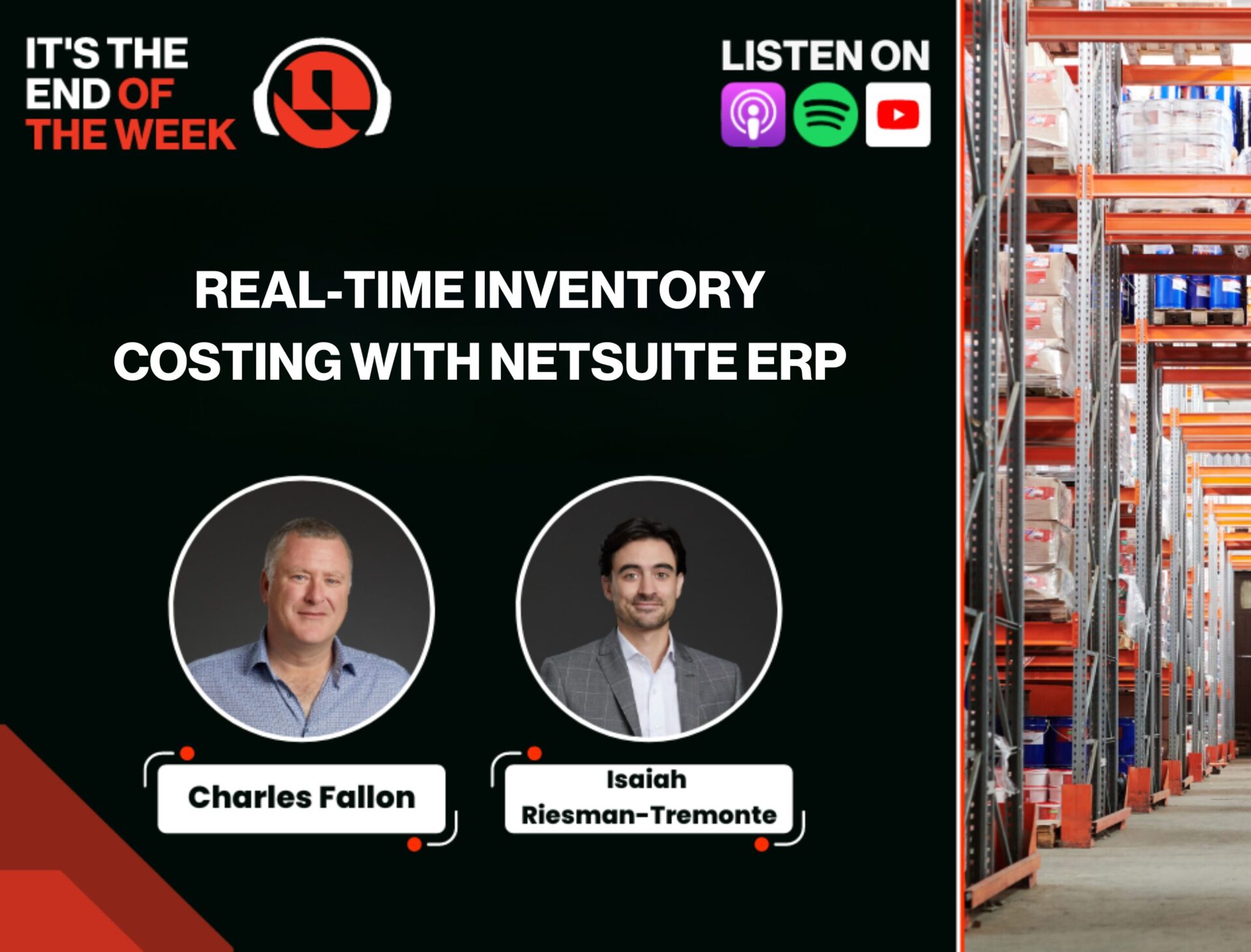
The Ins & Outs of Real-Time Inventory Costing
Master supply chain management with inventory cost accounting functionalities in NetSuite. Discover...
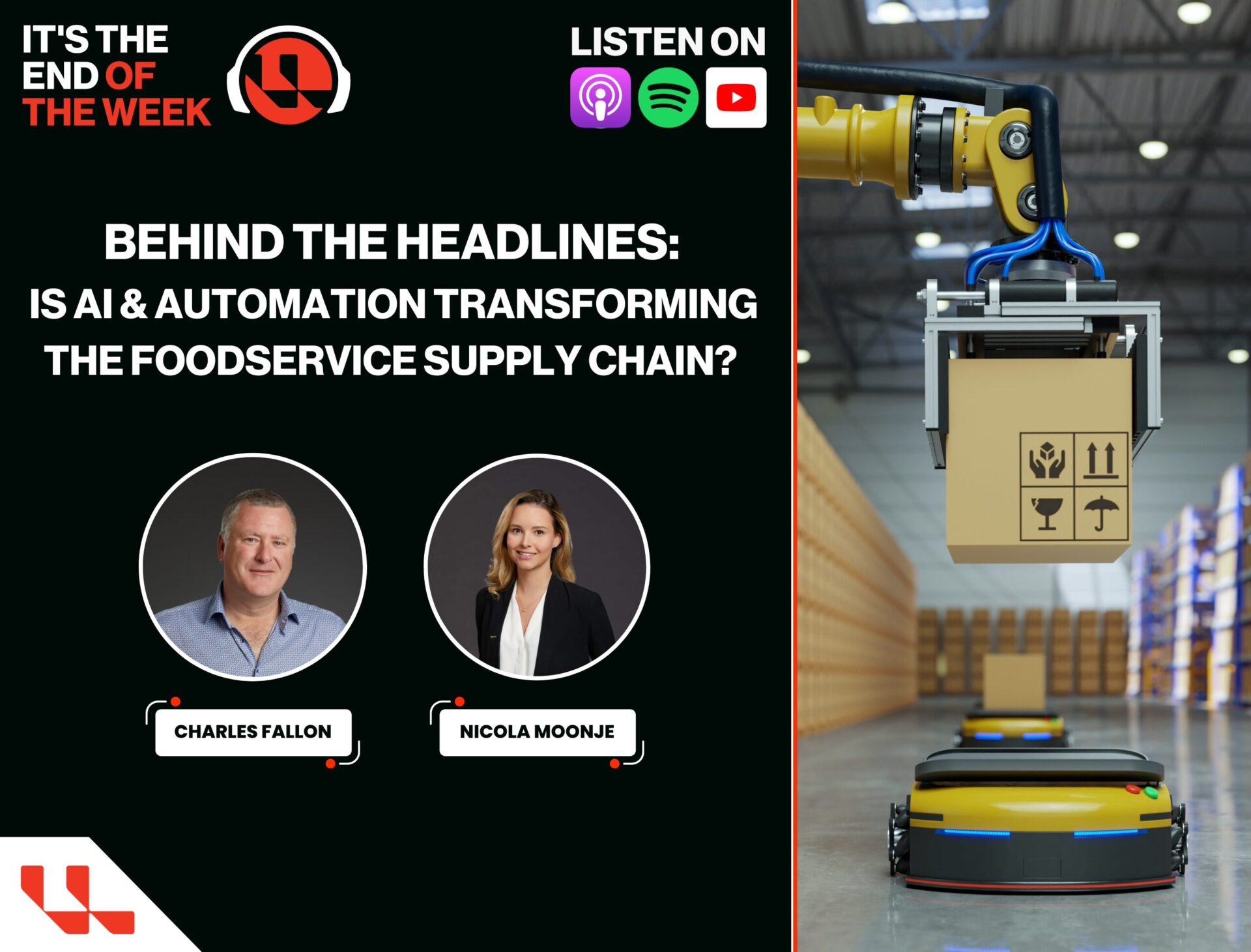
Will AI & Automation Transform the Foodservice Supply Chain?
The foodservice industry is undergoing a significant transformation, driven by advancements in AI an...
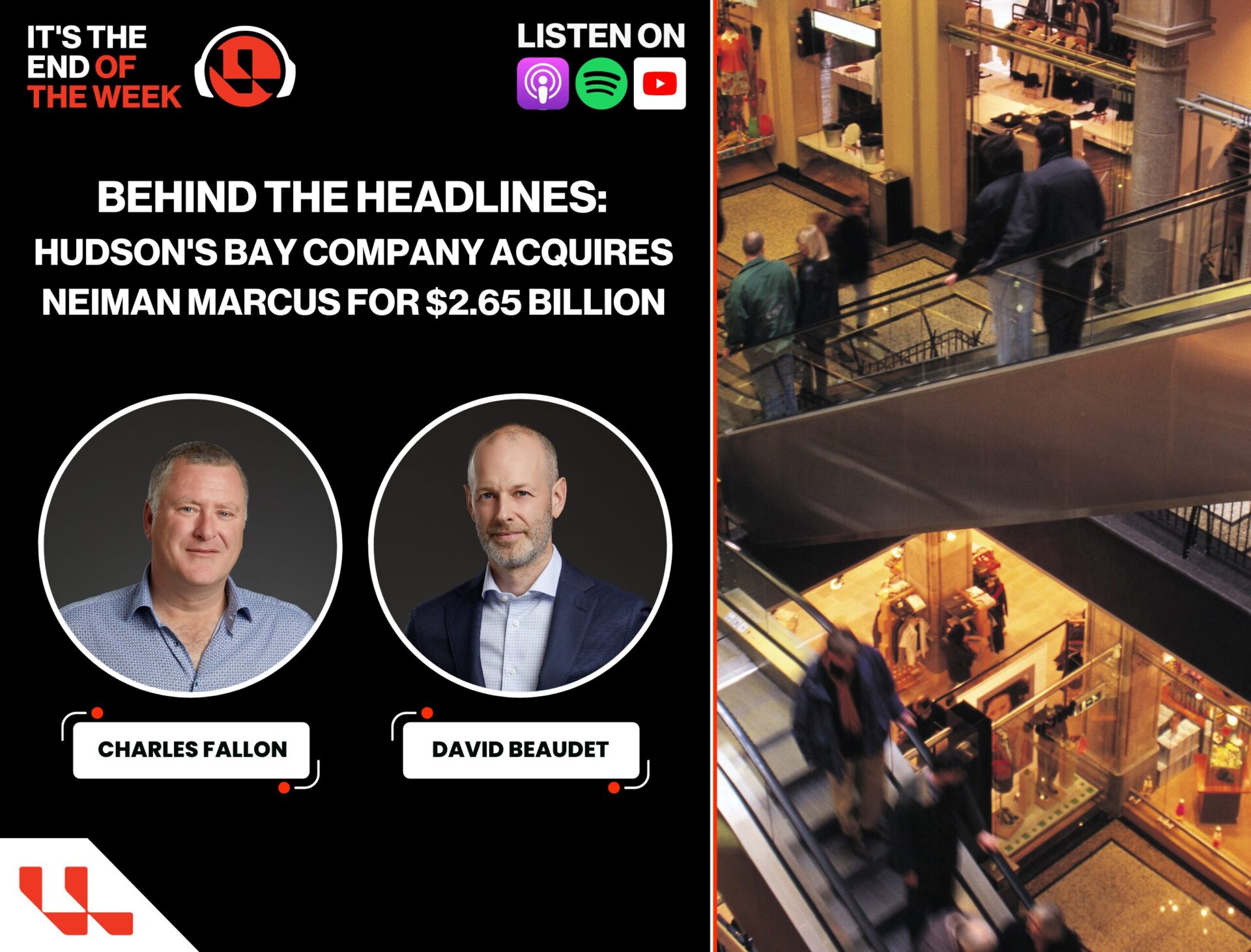
Behind the Headlines: Hudson’s Bay Company Acquires Neiman Marcus for $2.65 Billion
Hudson's Bay Company (HBC) makes waves in the luxury retail sector by acquiring Neiman Marcus, backe...
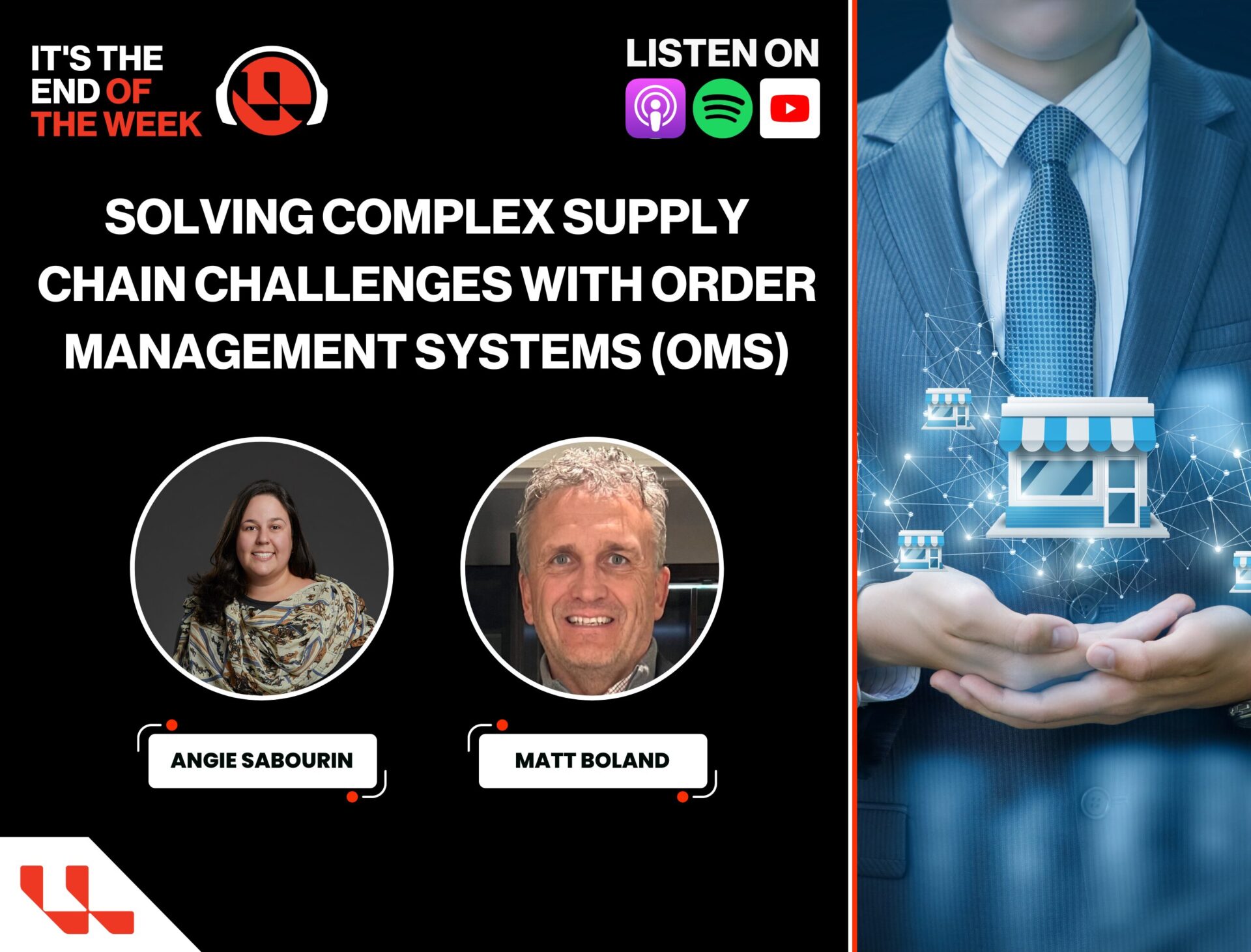
What Is an Order Management System (OMS) and How Can It Benefit Your Business?
The order management system (OMS) is a hot topic in supply chain - but what is it, and how can it be...
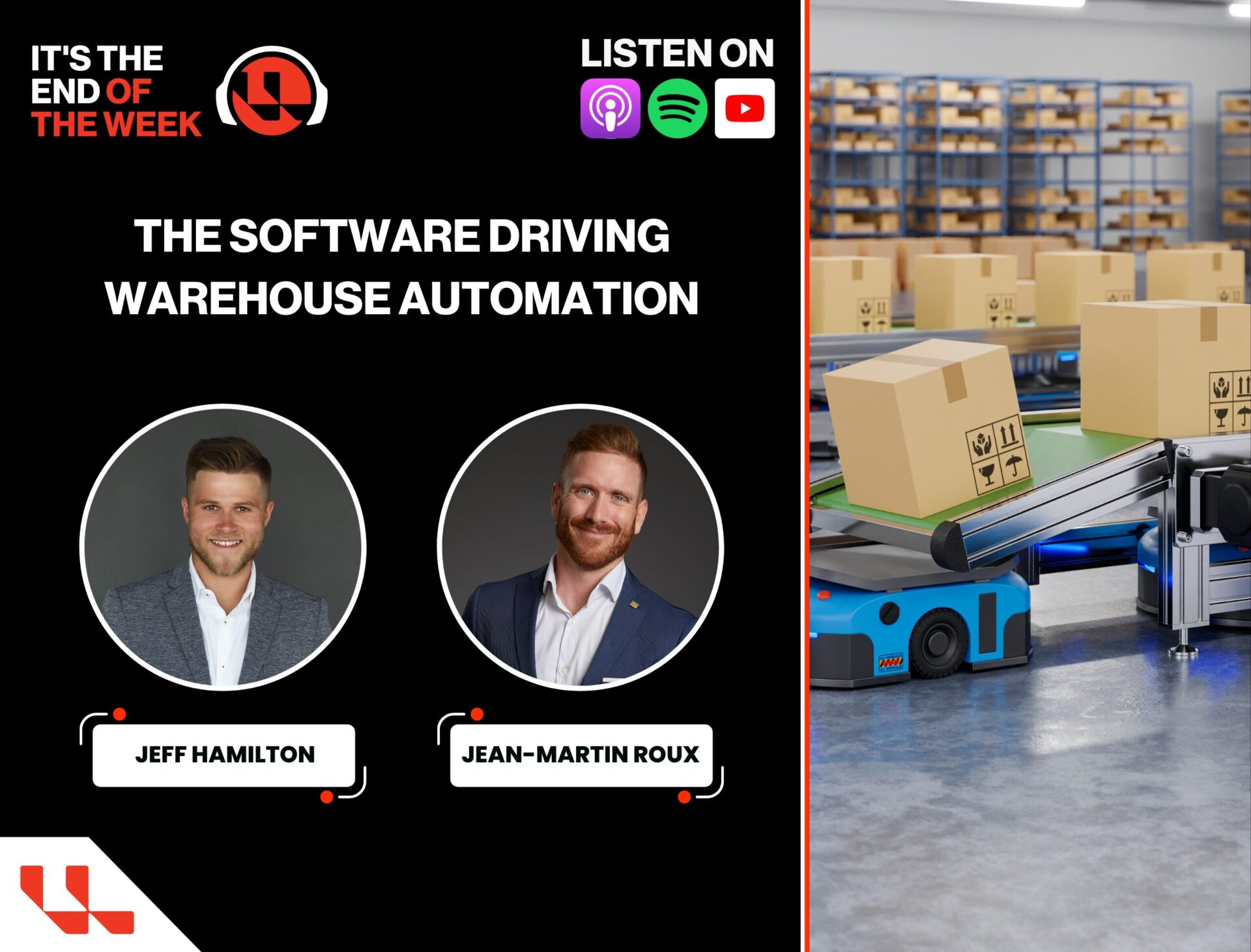
Warehouse Automation Software: A Deep Dive
Warehouse automation software is a critical component of modern supply chains, enabling businesses t...
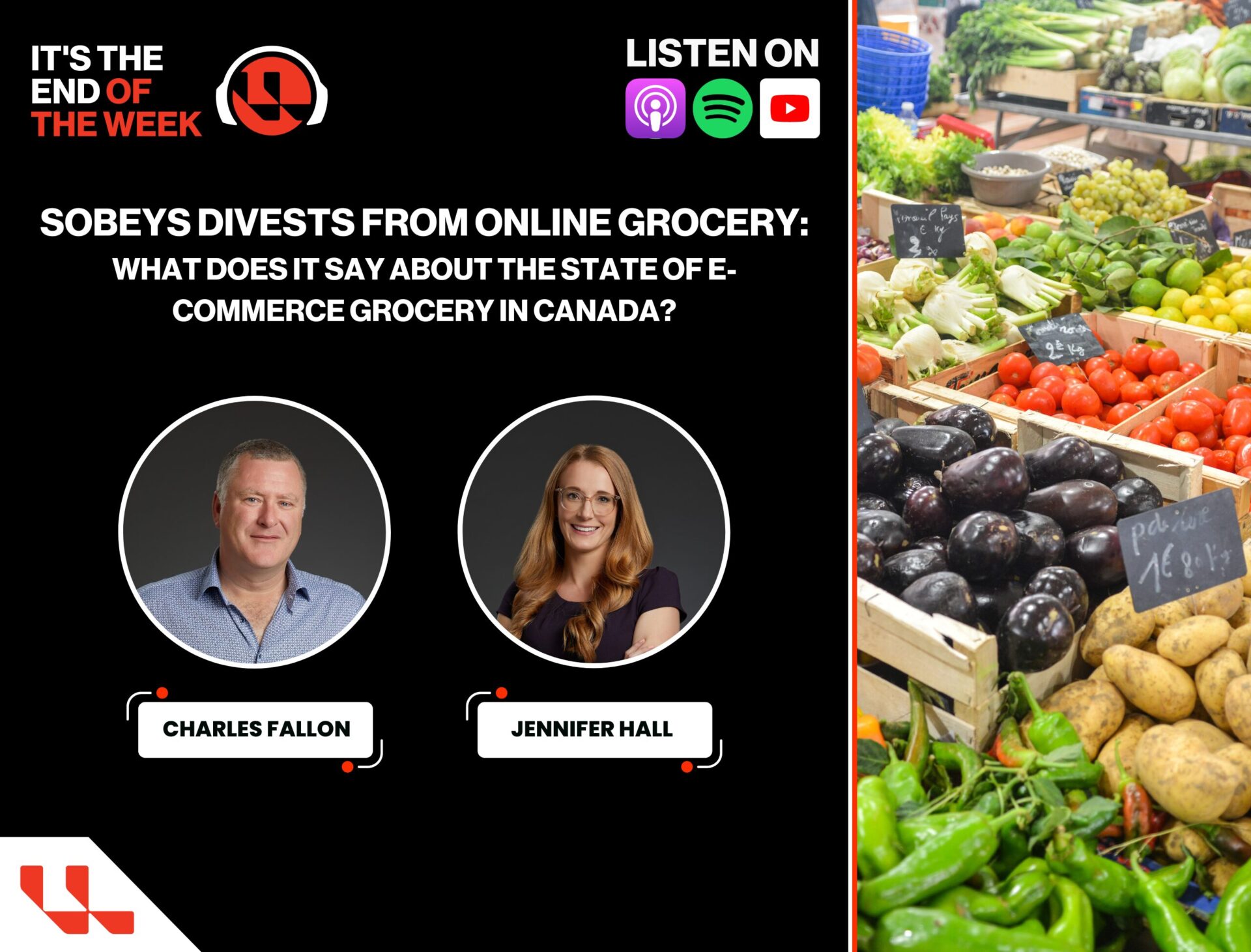
Sobeys’ E-Commerce Divestment: What Does It Mean for the Future of Online Grocery in Canada?
Sobeys' surprising split with Ocado signals a shift in the Canadian grocery landscape. Explore the i...
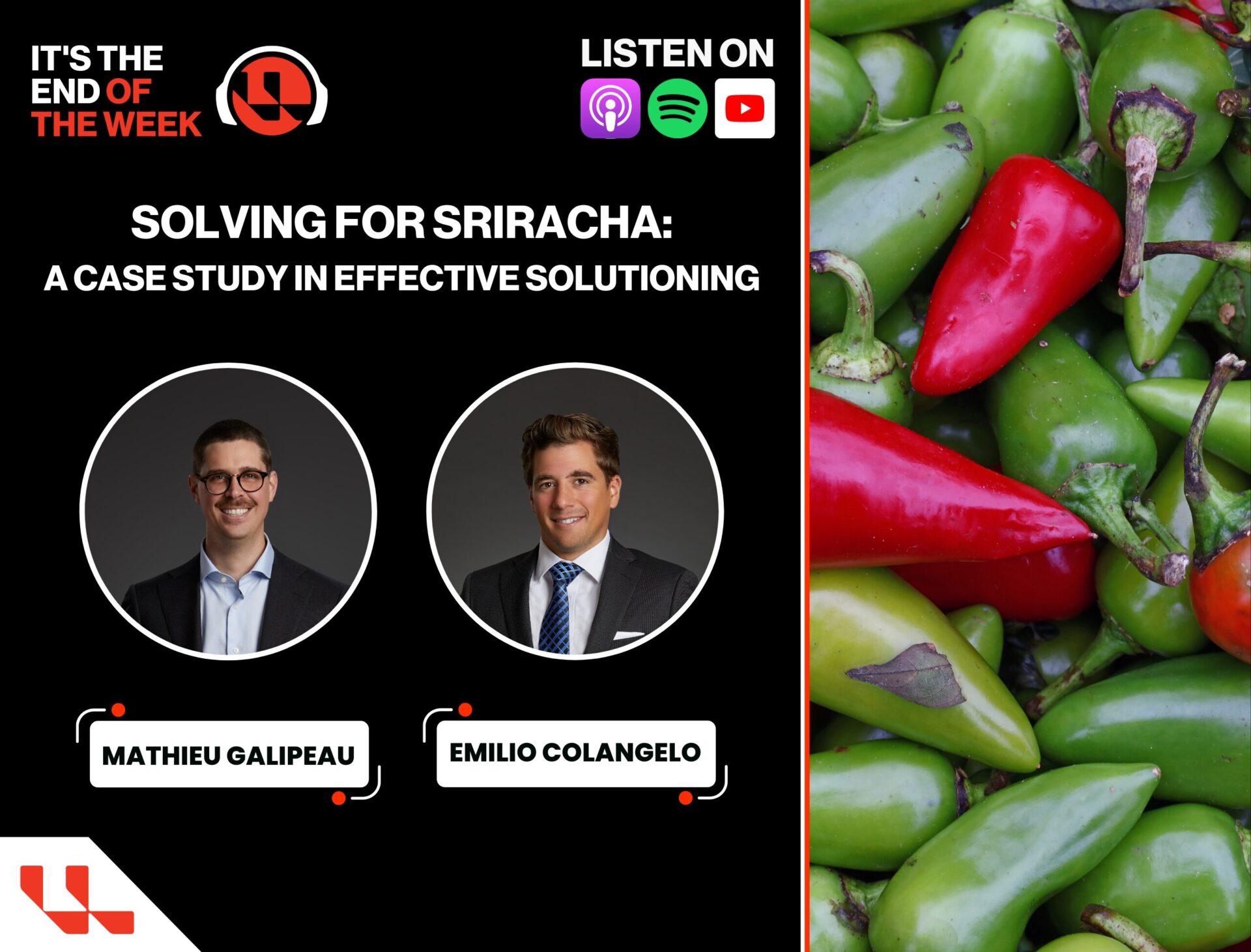
Solving For Sriracha: A Case Study in Effective Solutioning
Nationwide, there is a sriracha shortage. What's going on? And what can this spicy situation teach u...
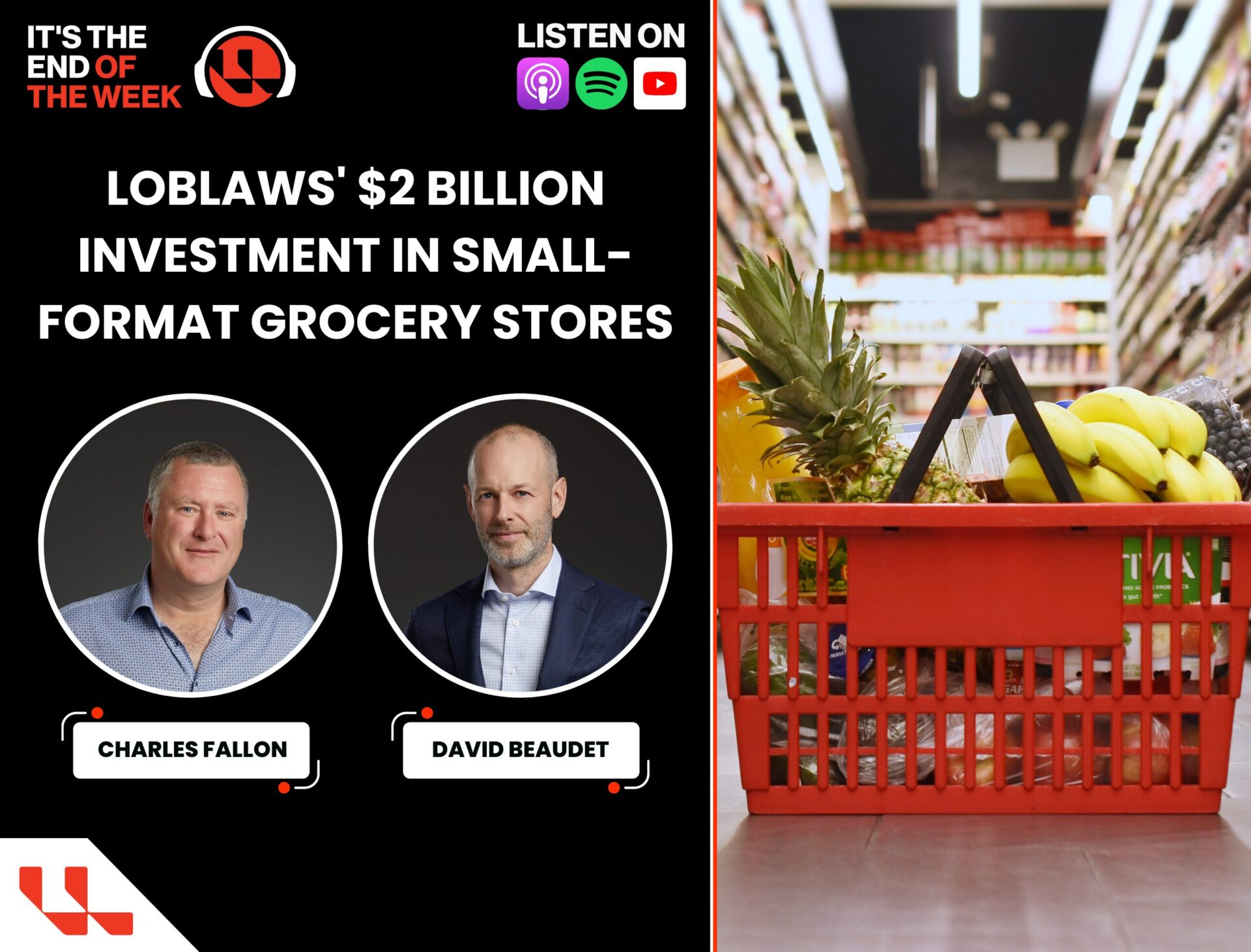
The Implications of Loblaws’ $2 Billion Small-Format Grocery Store Play
Loblaws announces a $2 billion investment in small-format discount stores across Canada - is it the...
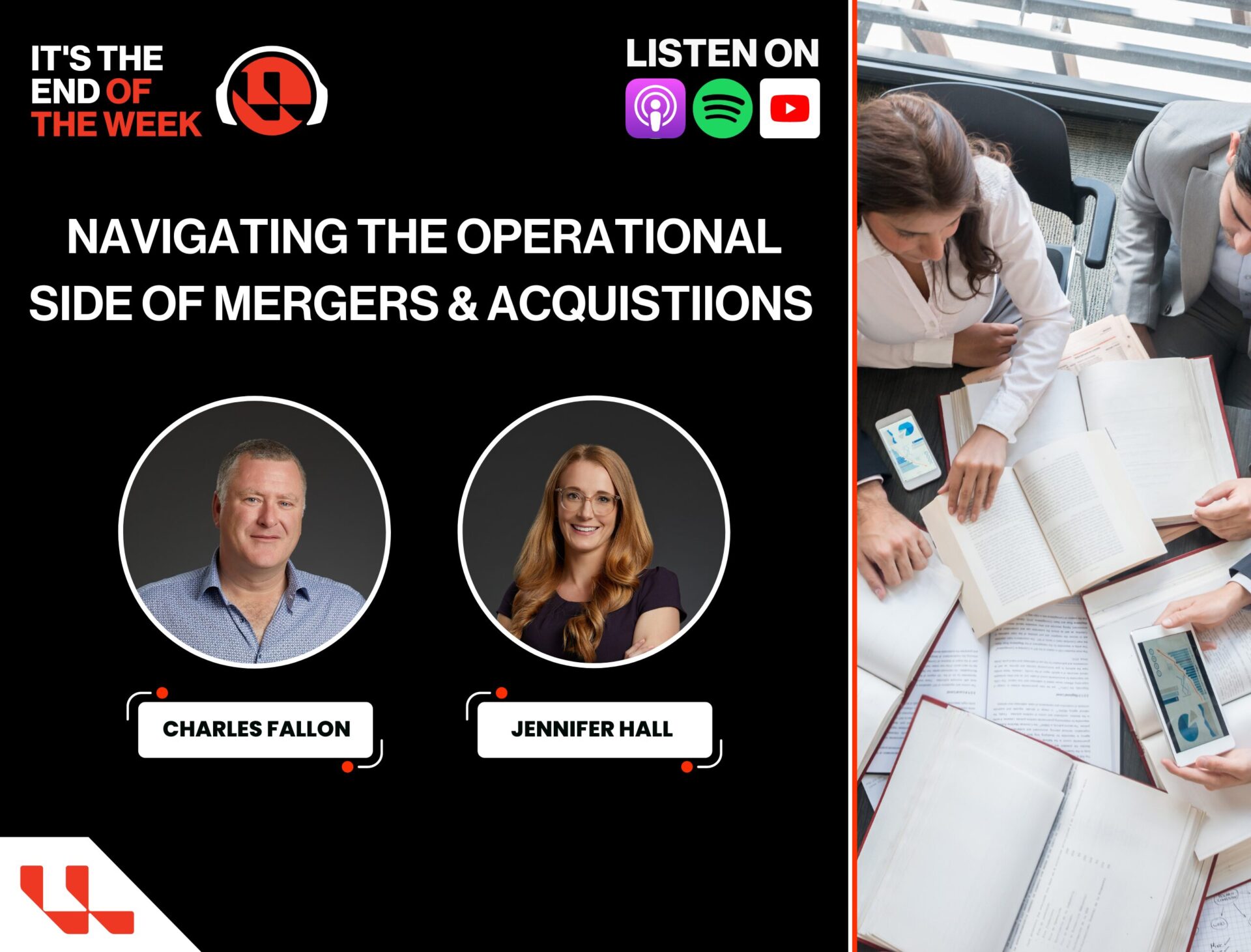
Navigating the Operational Side of Mergers and Acquisitions
Mergers and acquisitions (M&A) represent a dynamic and crucial facet of the business world. This blo...
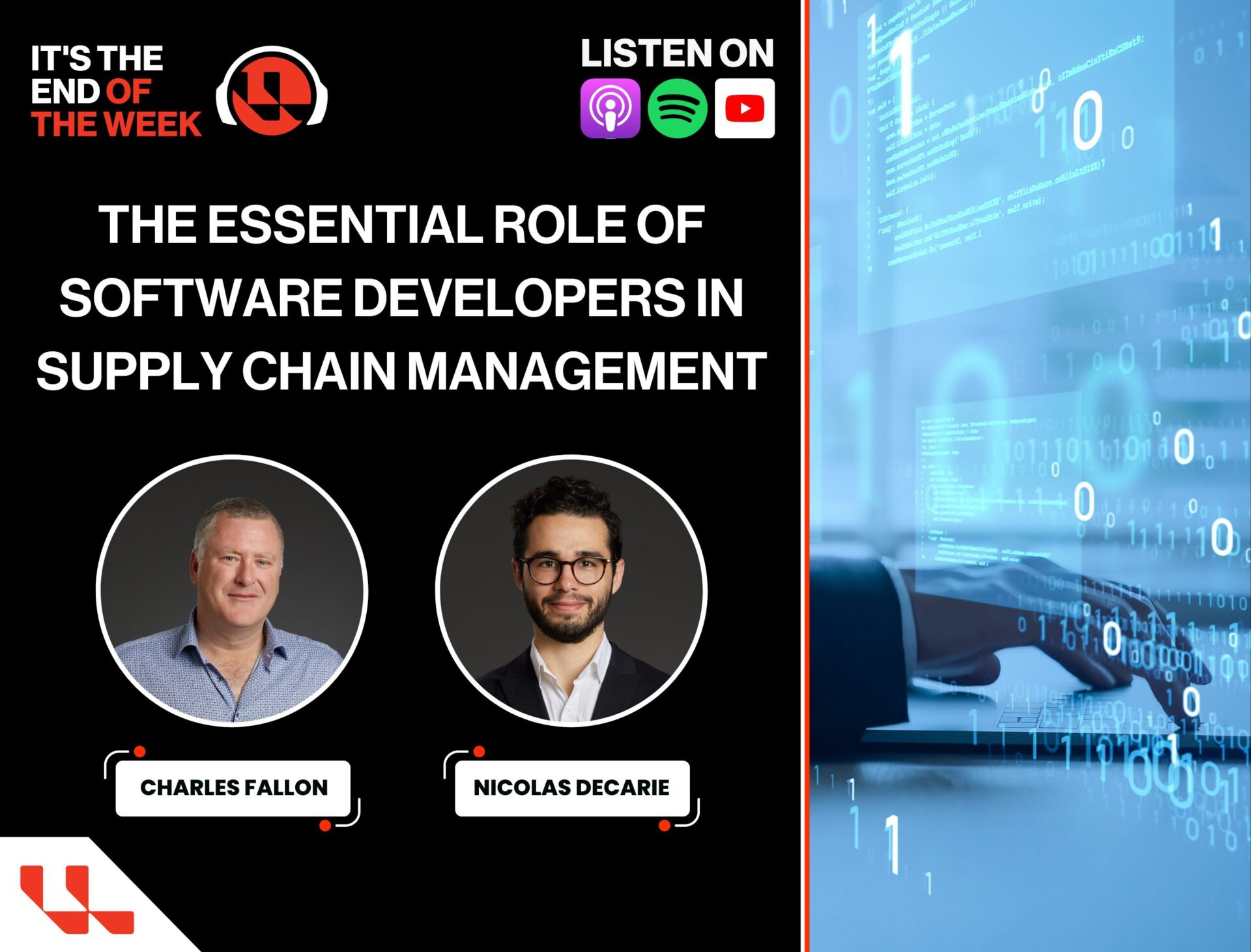
The Role of Software Development in Supply Chain Management
Software development is pivotal in supply chain management, providing tailored solutions that enhanc...
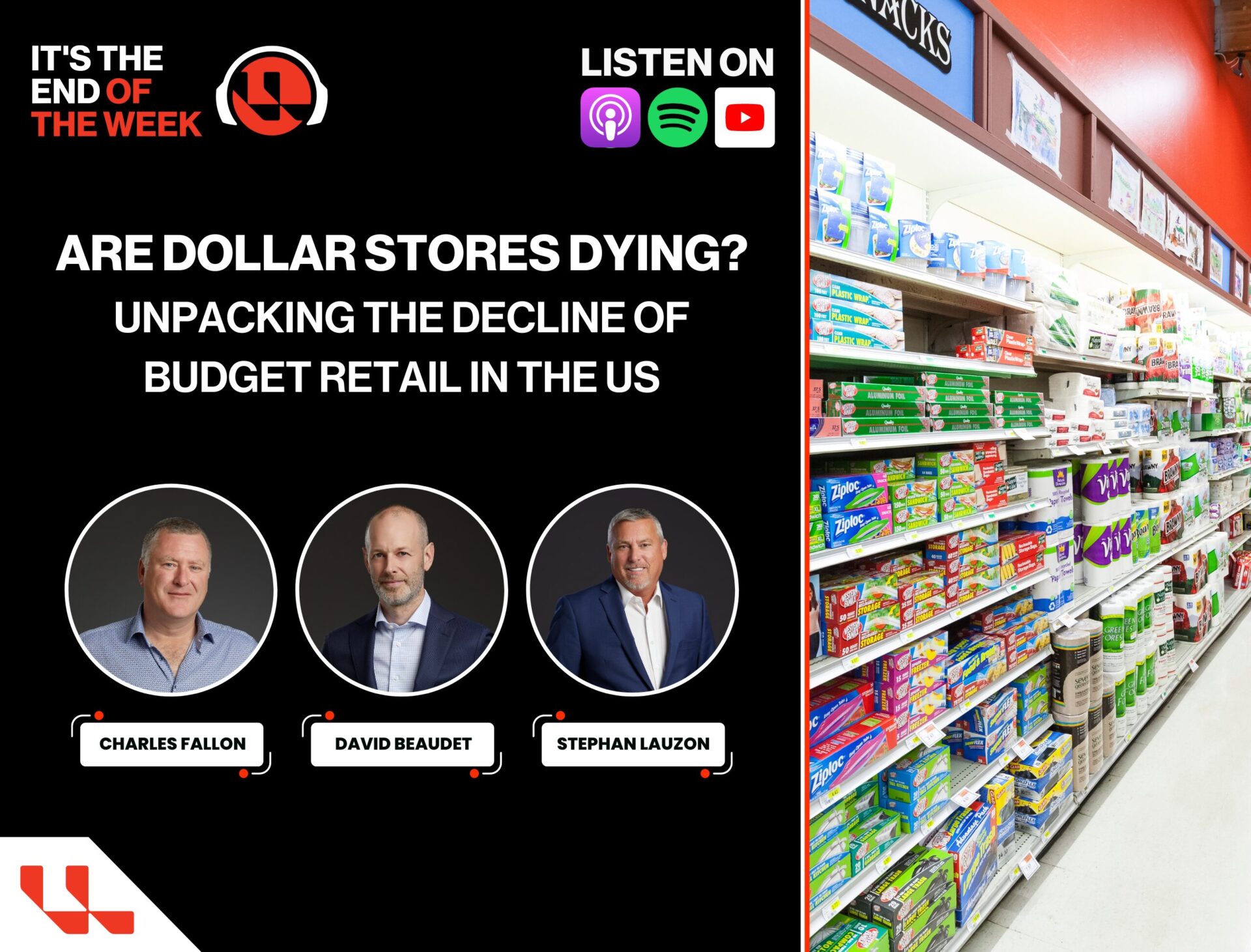
Unpacking the Decline of Dollar Stores in America
Take a deep dive into the pros and cons of Natural Language Processing (NLP) and it's application in...
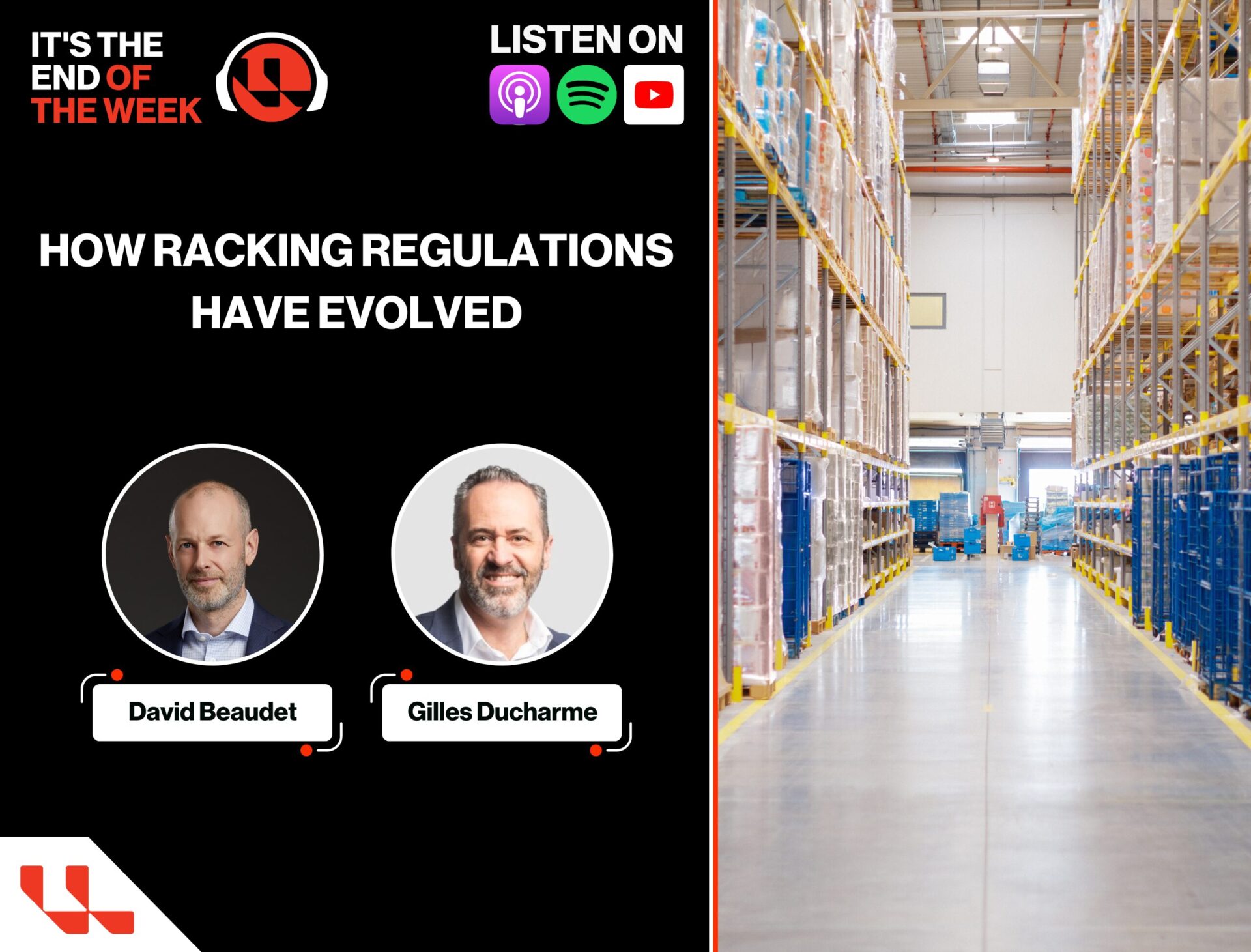
How Racking Regulations Have Evolved
Take a deep dive into the pros and cons of Natural Language Processing (NLP) and it's application in...
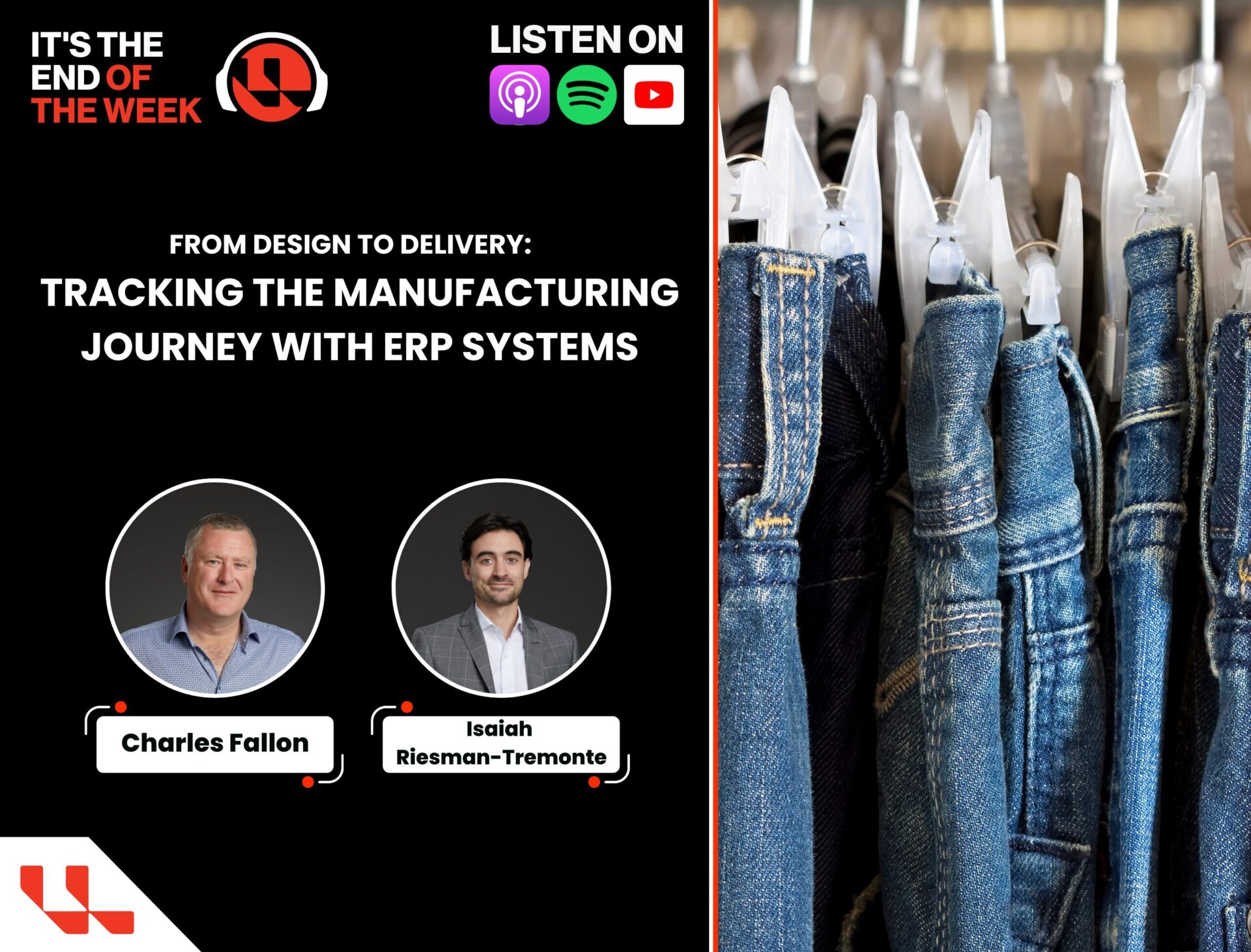
Tracking the Manufacturing Journey with ERP Systems
Take a deep dive into the pros and cons of Natural Language Processing (NLP) and it's application in...
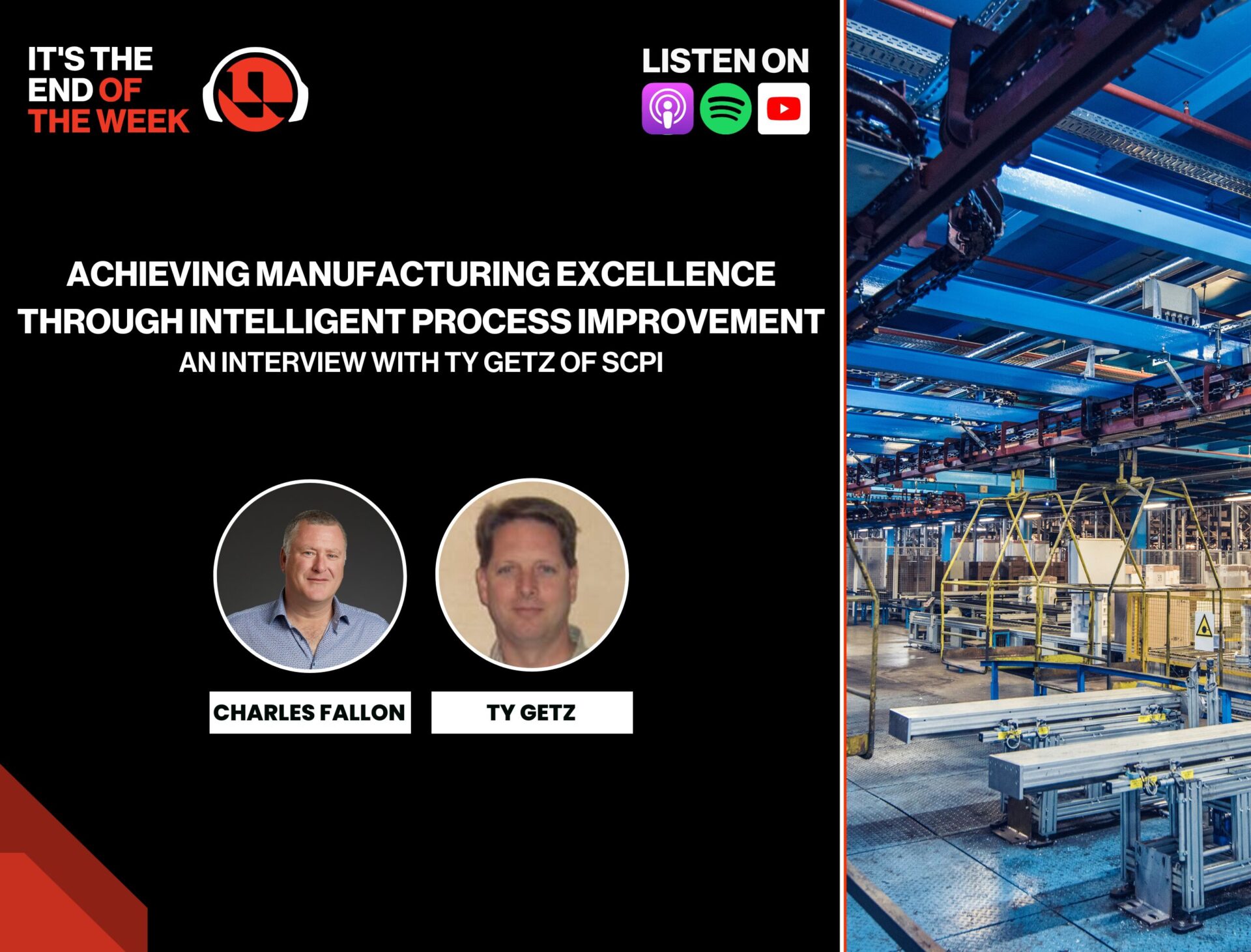
How Manufacturers Navigate From Push to Pull Operations
Take a deep dive into the pros and cons of Natural Language Processing (NLP) and it's application in...
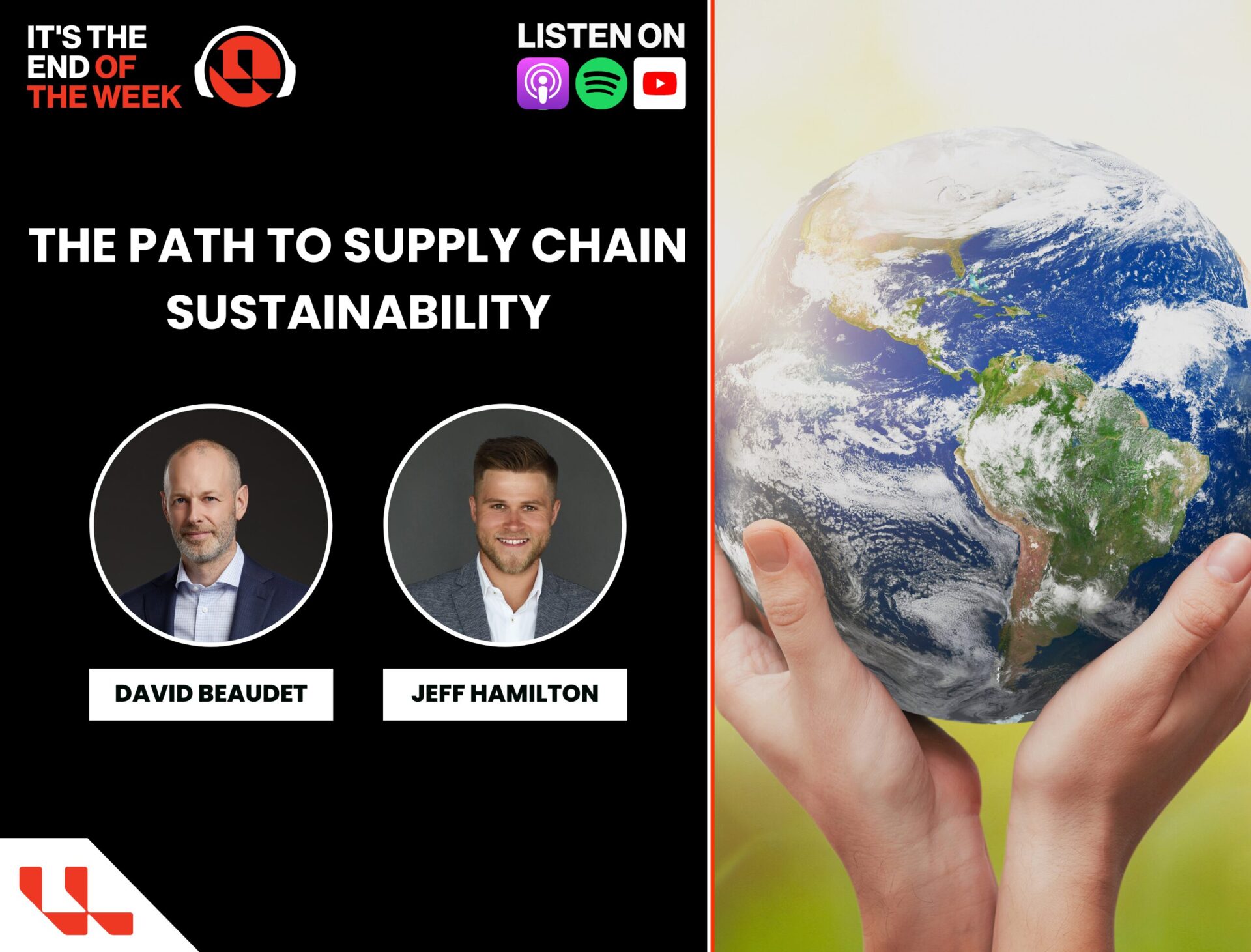
The Path to Supply Chain Sustainability
Take a deep dive into the pros and cons of Natural Language Processing (NLP) and it's application in...
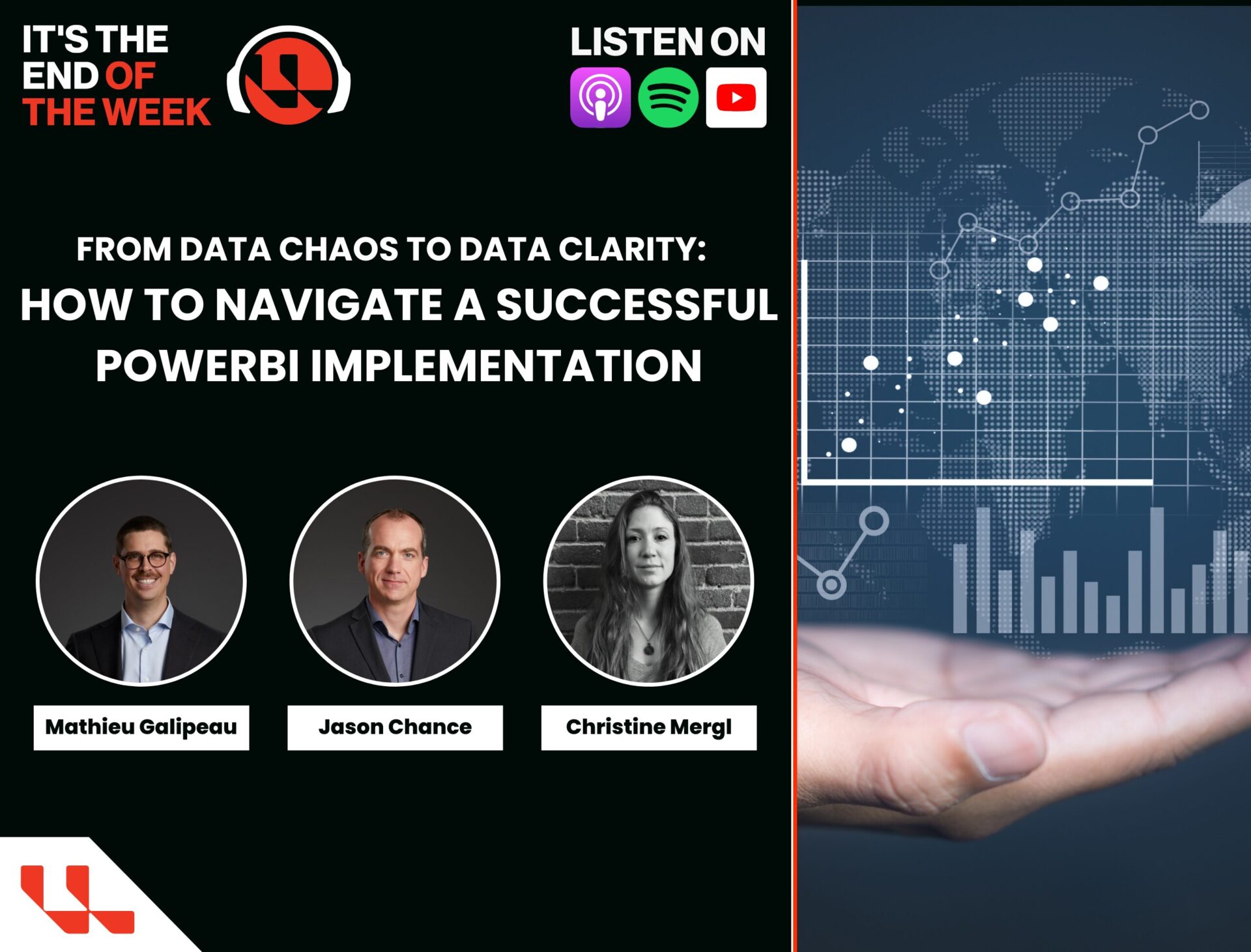
From Data Chaos to Data Clarity: How to Navigate a Successful PowerBI Implementation
Take a deep dive into the pros and cons of Natural Language Processing (NLP) and it's application in...
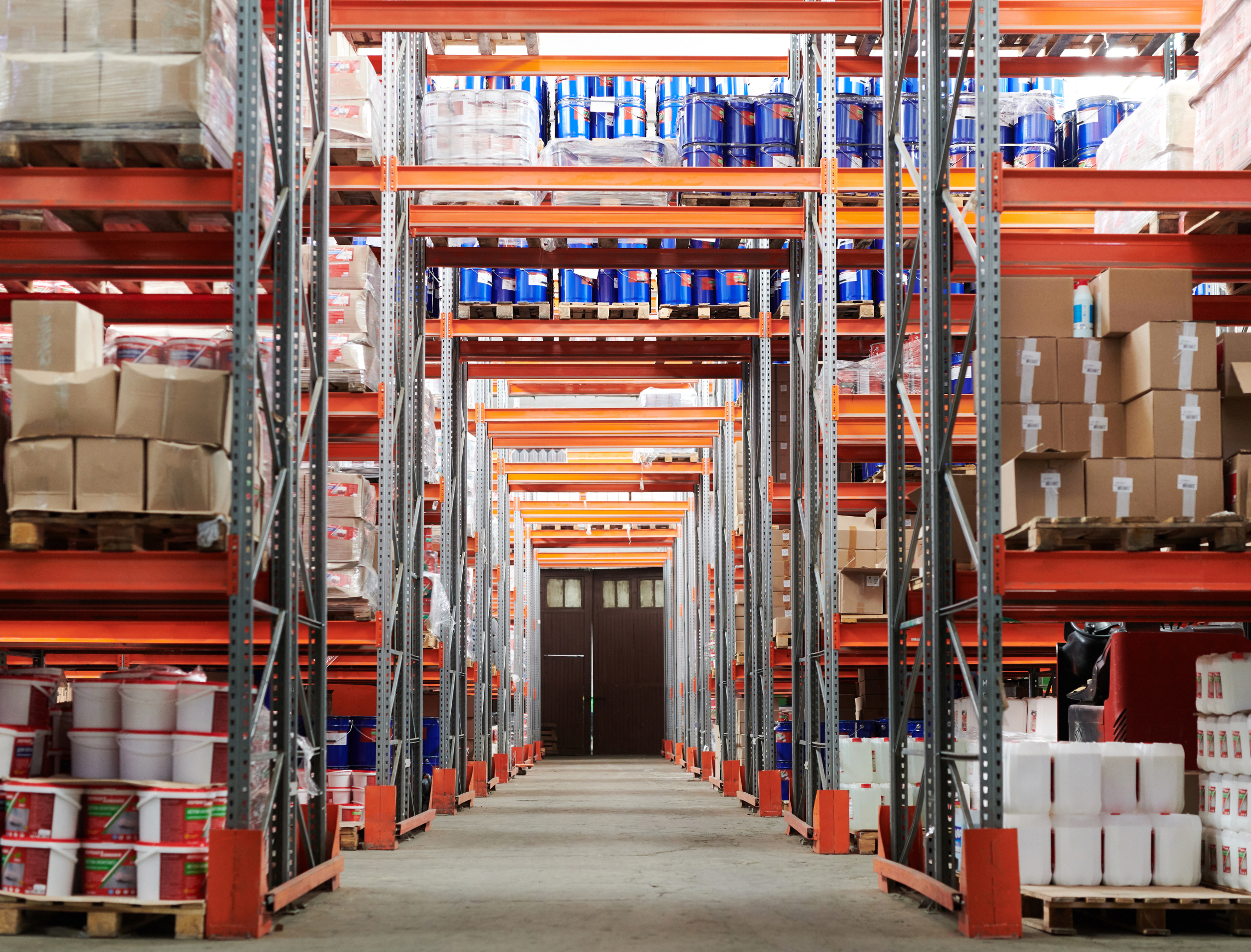
The Keys to Successful Warehouse Implementation
In the complex landscape of supply chain management, physical infrastructure serves as the backbone...
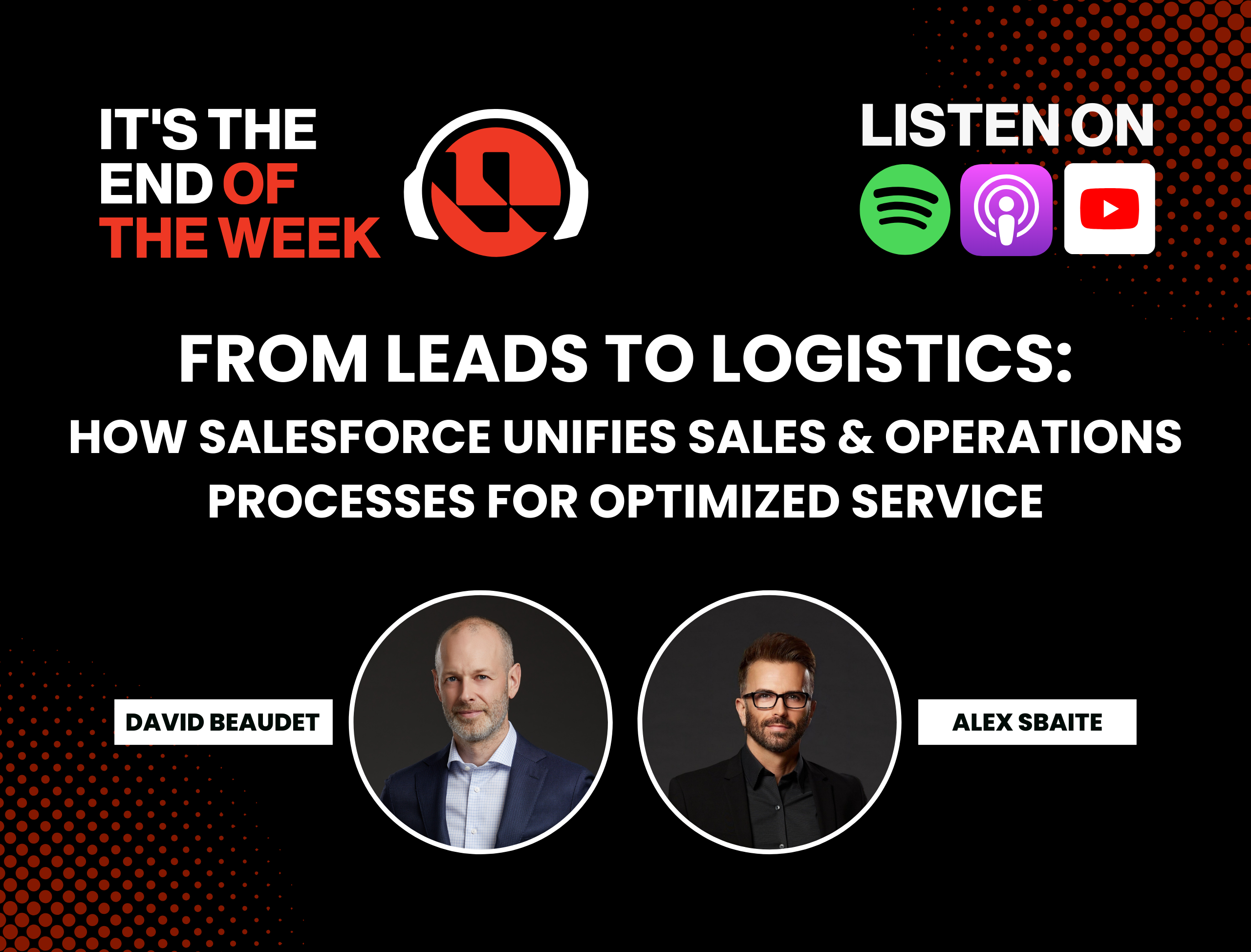
From Leads to Logistics: How Salesforce Unifies Sales & Operations Processes for Optimal Service
It's the end of the week and our hosts are joined by Danny Wang to discuss data visualization. Busin...
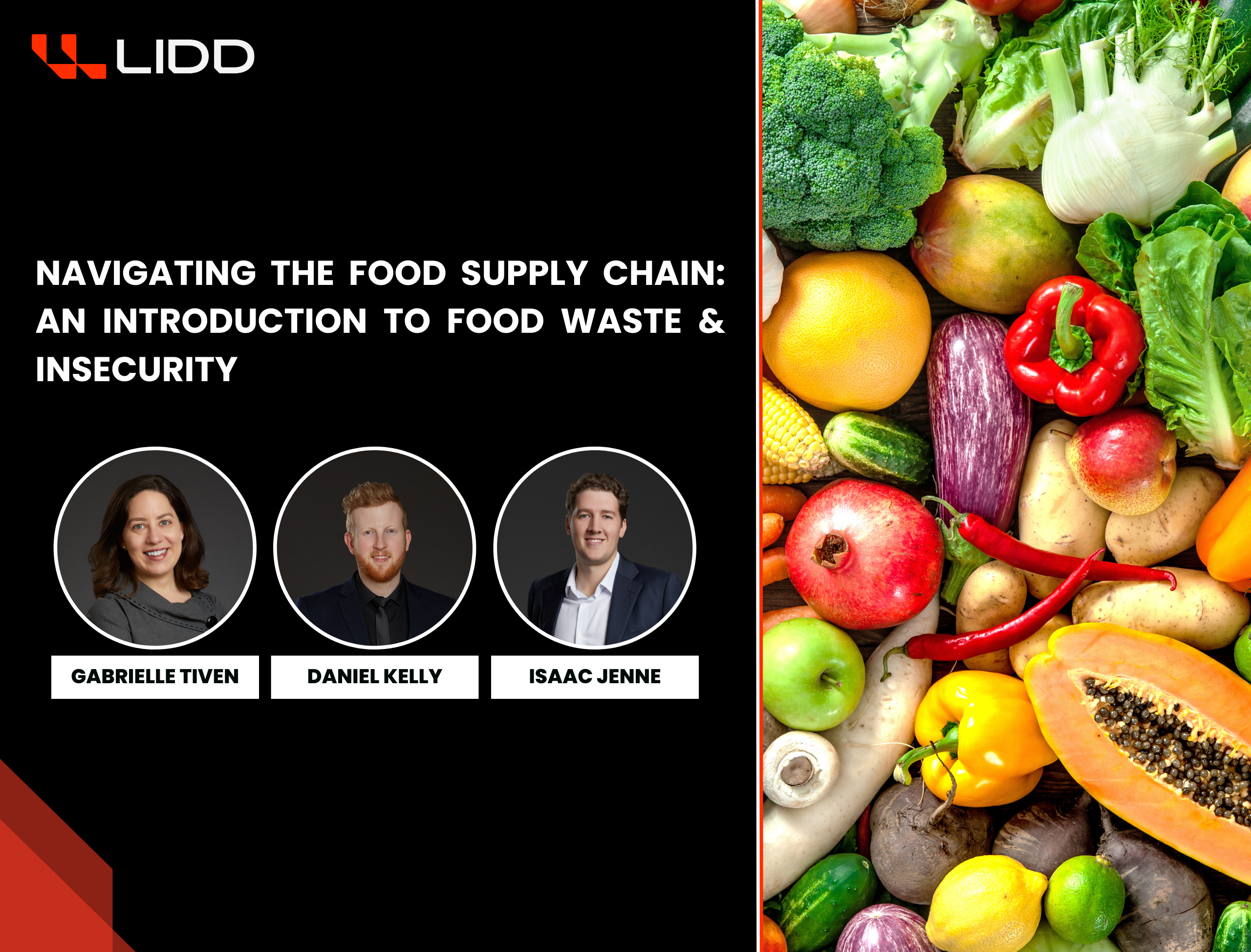
Addressing Food Waste and Insecurity in the North American Supply Chain
Get insights on how LIDD, Food Banks Canada, and the World Wildlife Fund are working together to hel...
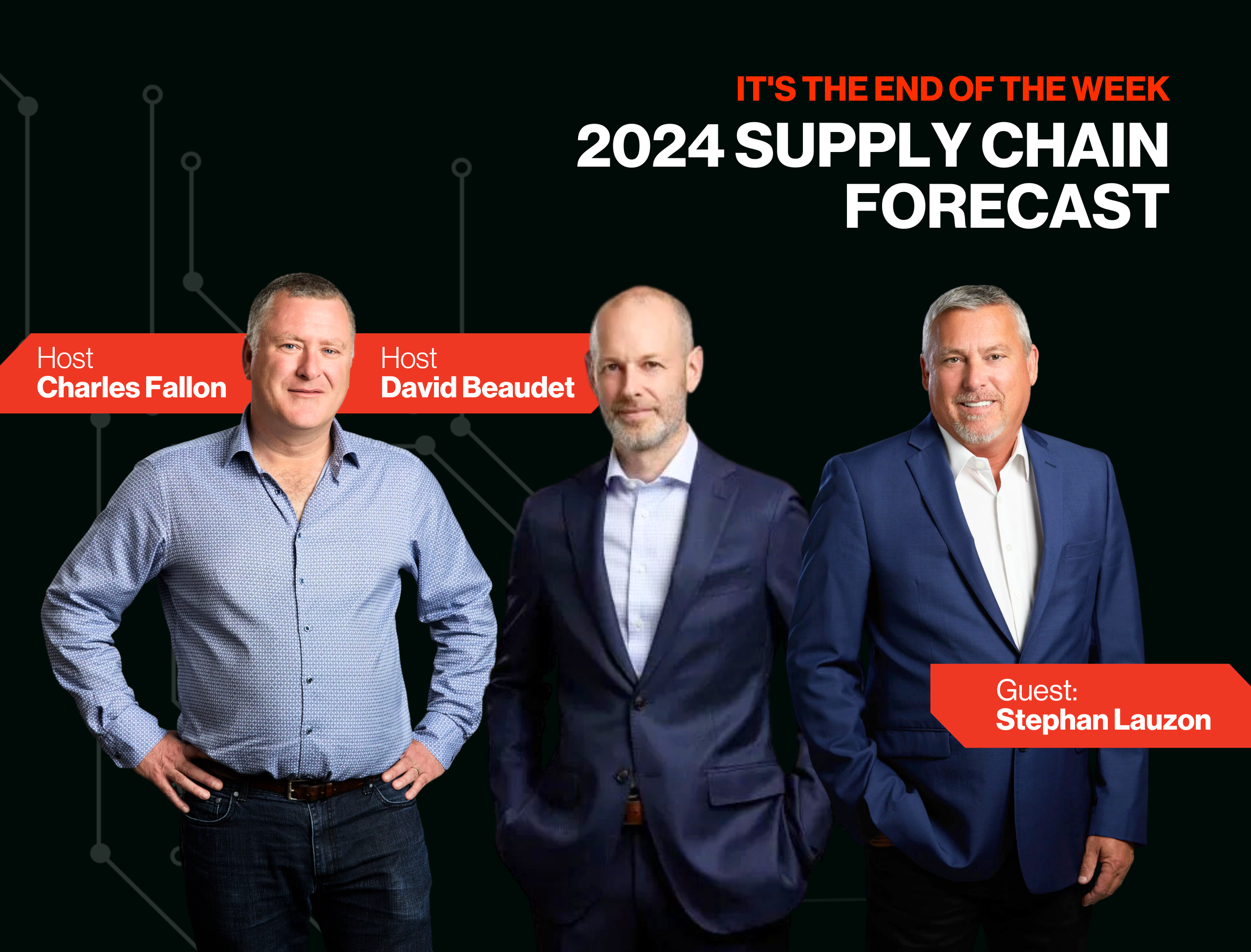
2024 Supply Chain Forecast: AI, Machine Customers & ESG
It's the end of the week and our hosts are joined by Danny Wang to discuss data visualization. Busin...
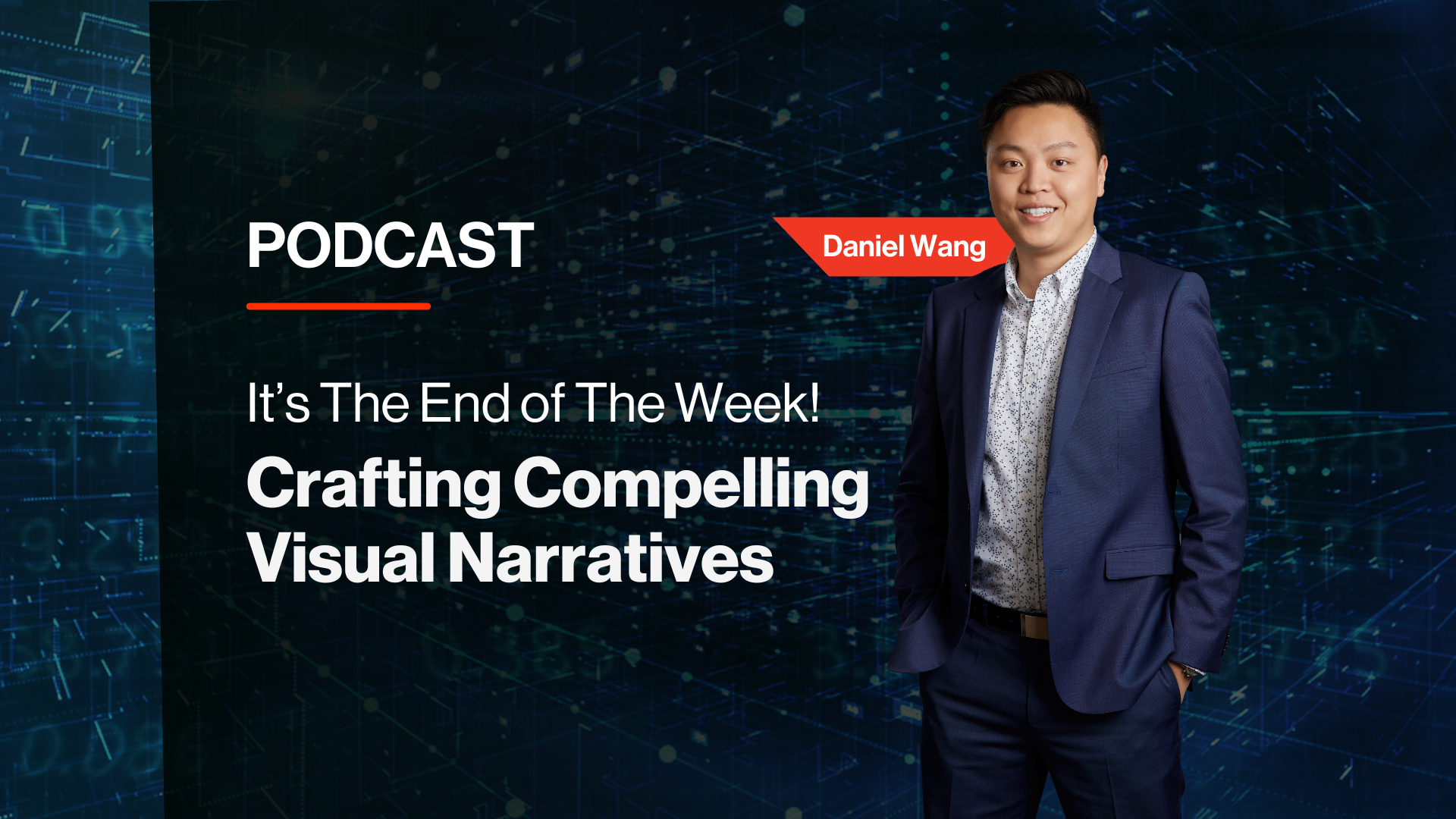
Crafting Compelling Visual Narratives: How to Improve Your Presentations
It's the end of the week and our hosts are joined by Danny Wang to discuss data visualization. Busin...
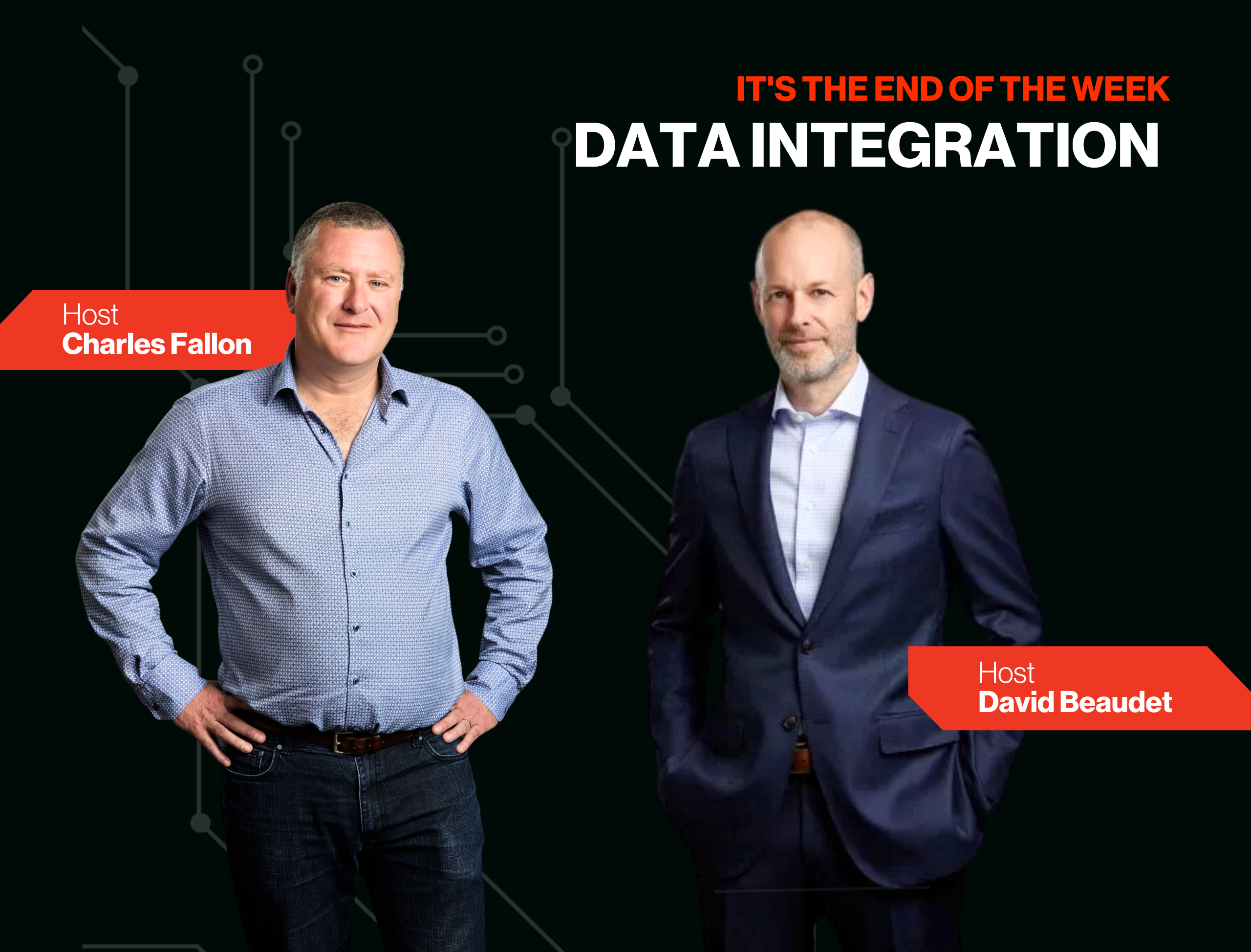
Exploring Integrations – The History of FTP and the Modern Day Applications for SOAP & REST APIs
It's the end of the week and our hosts are joined by Sabrina Sasu to discuss data integration. Data...
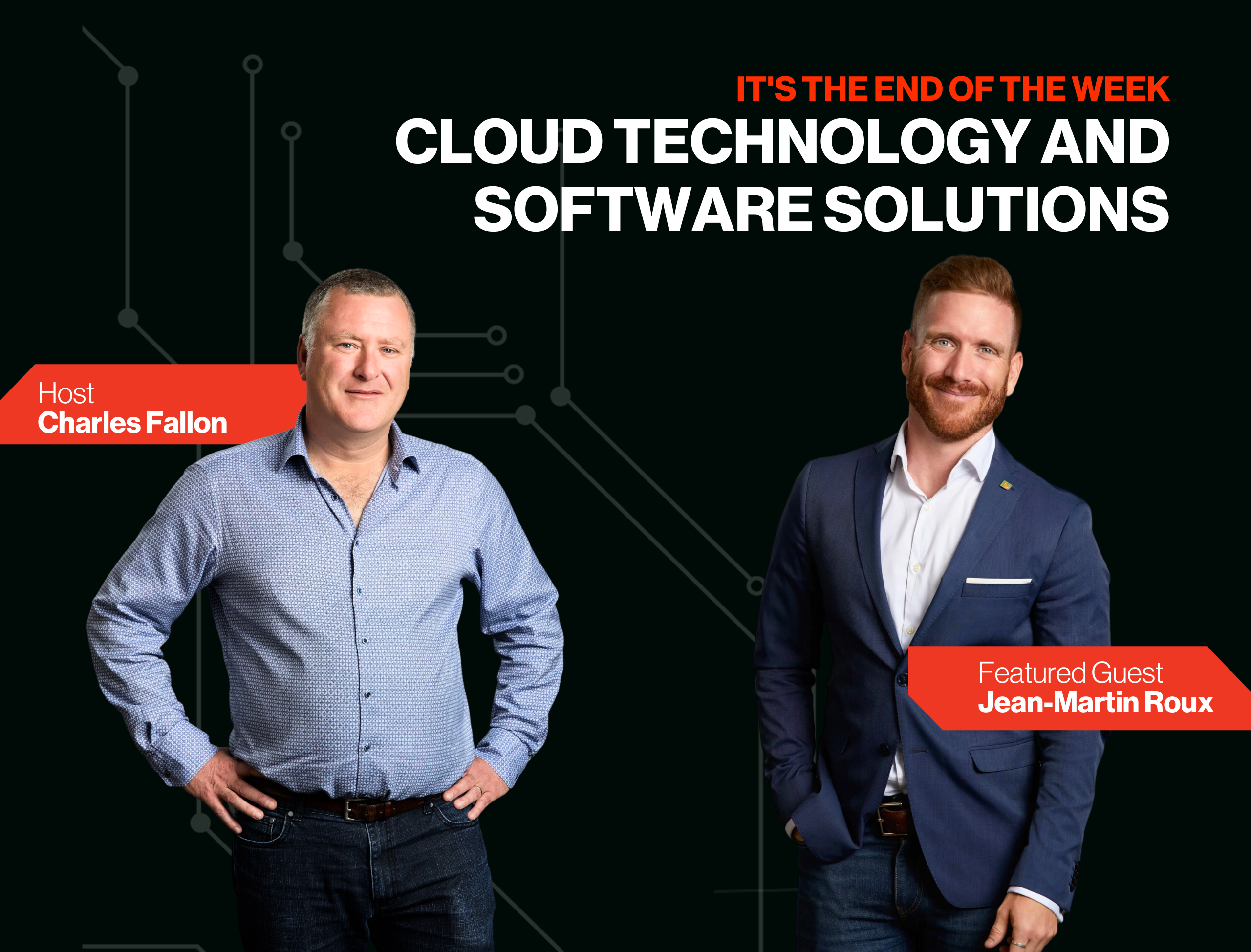
Mastering the Cloud – WMS Technology, Key Players and Factors to Consider
Its *The Start of the Week* and we are joined by Charles Fallon and Jean-Martin Roux for another epi...
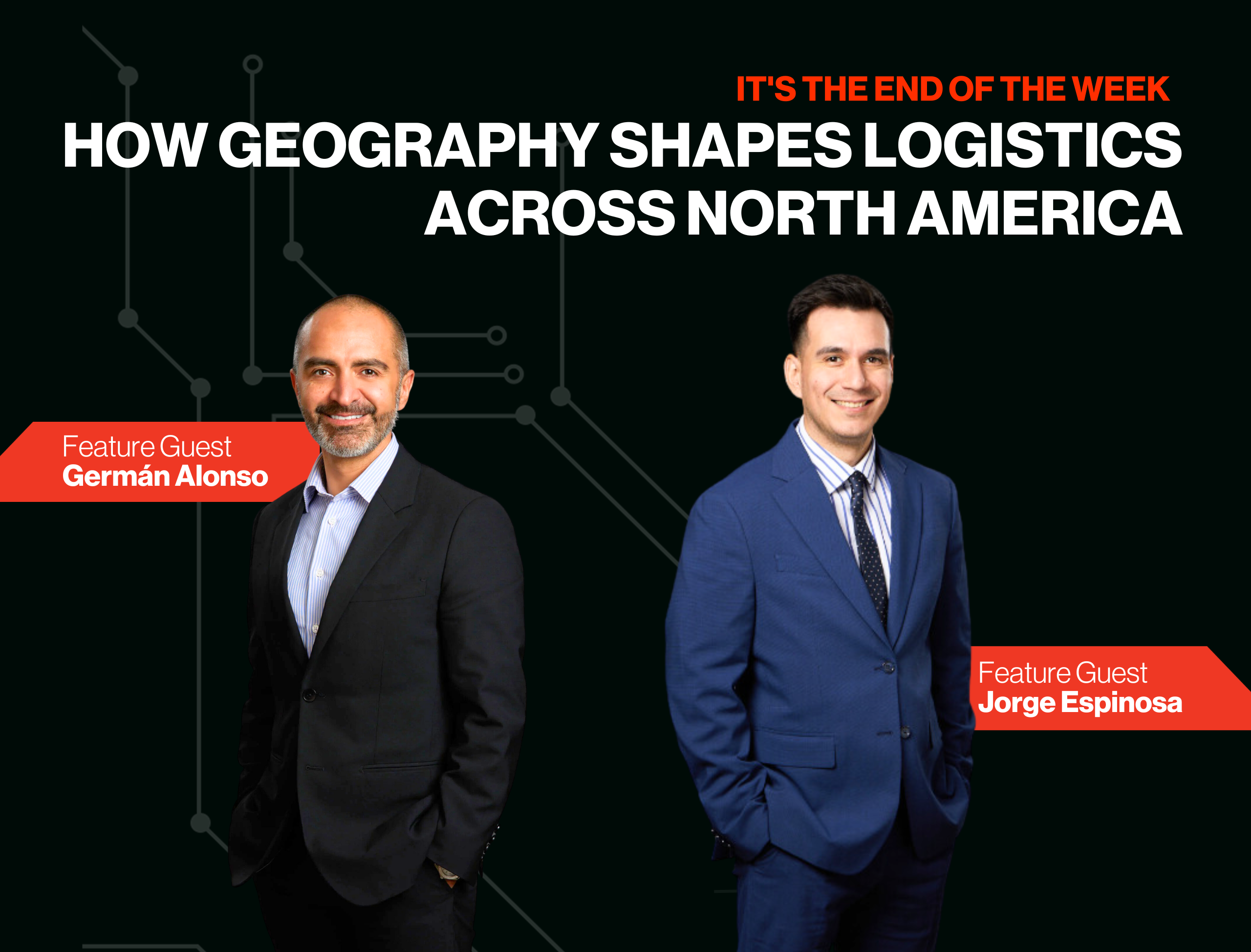
How Geography Shapes Logistics Across North America – It’s the End of the Week!
On this week's episode of It's the End of the Week, our hosts knead through the operational strategi...
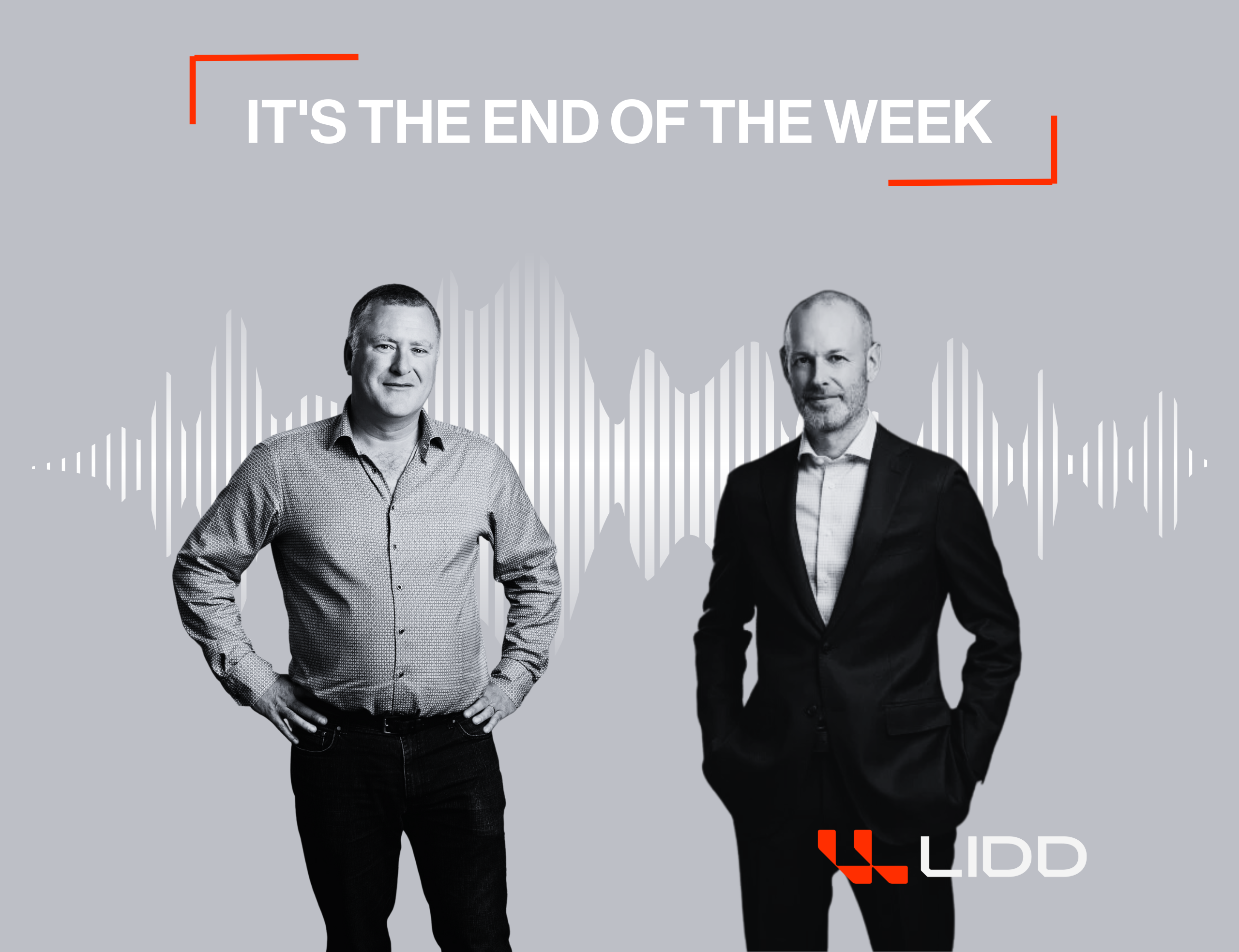
Dominos’ Pizza Preeminence: Insights into the Supply Chain of the World’s Largest Pizza Chain
On this week's episode of It's the End of the Week, our hosts knead through the operational strategi...
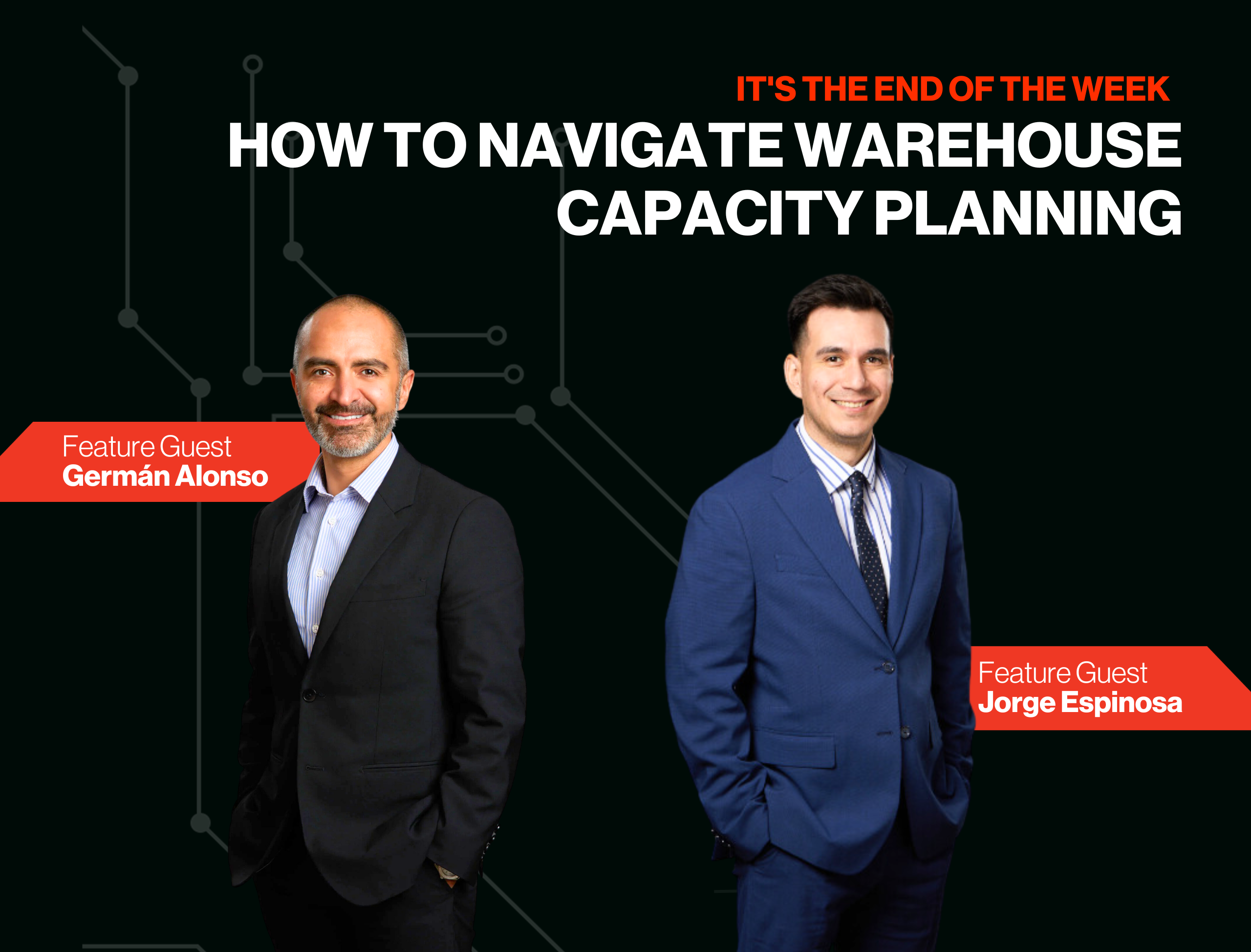
How to Navigate Warehouse Capacity Planning? – It’s the End of the Week!
Join Germán and Jorge on our first-ever Spanish episode of "It's the End of the Week" as they discus...
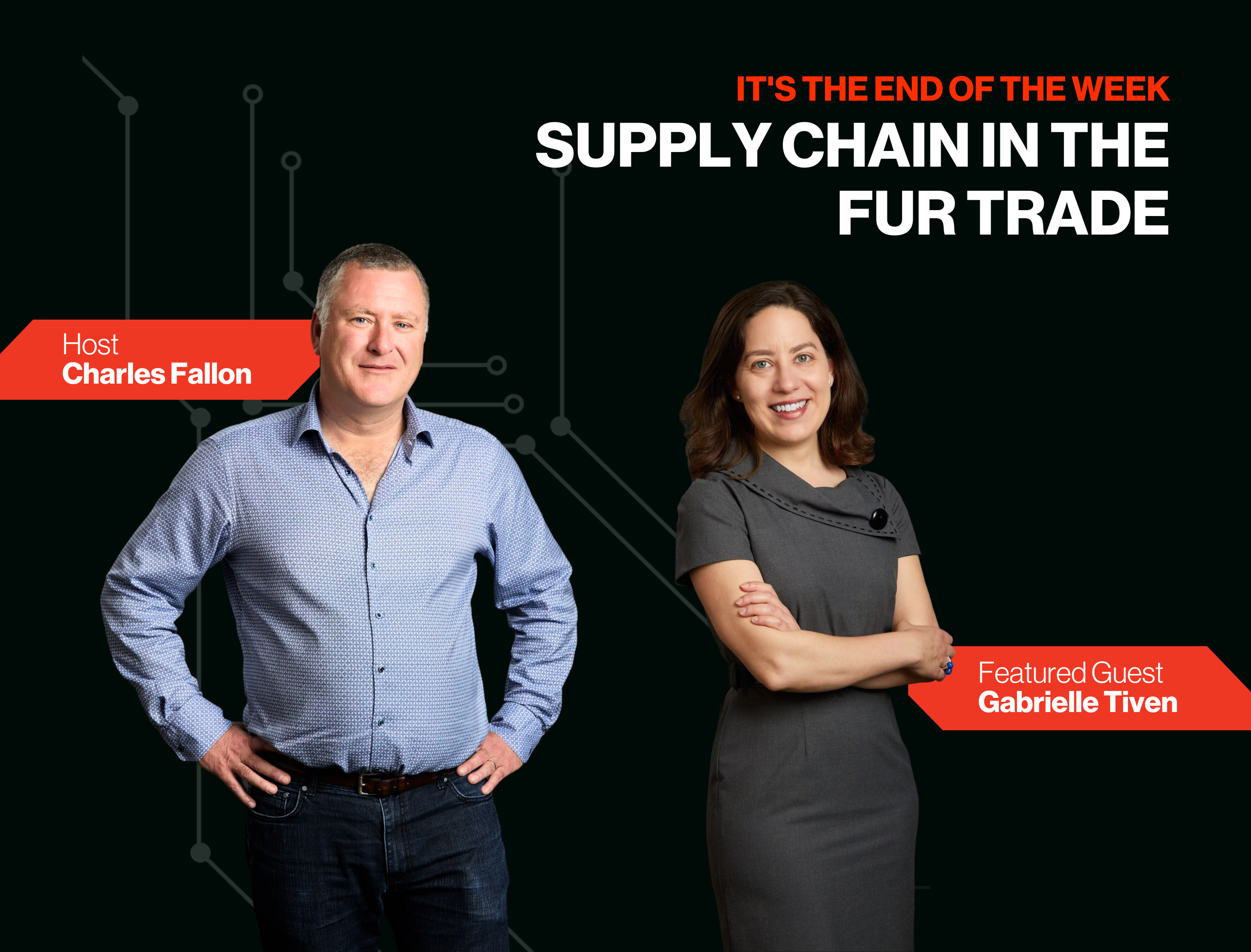
Supply Chain Engineering in the Fur Trade – It’s the End of the Week!
Gabrielle Tiven and Charles Fallon explore how the age-old strategies of the Canadian fur trade prov...
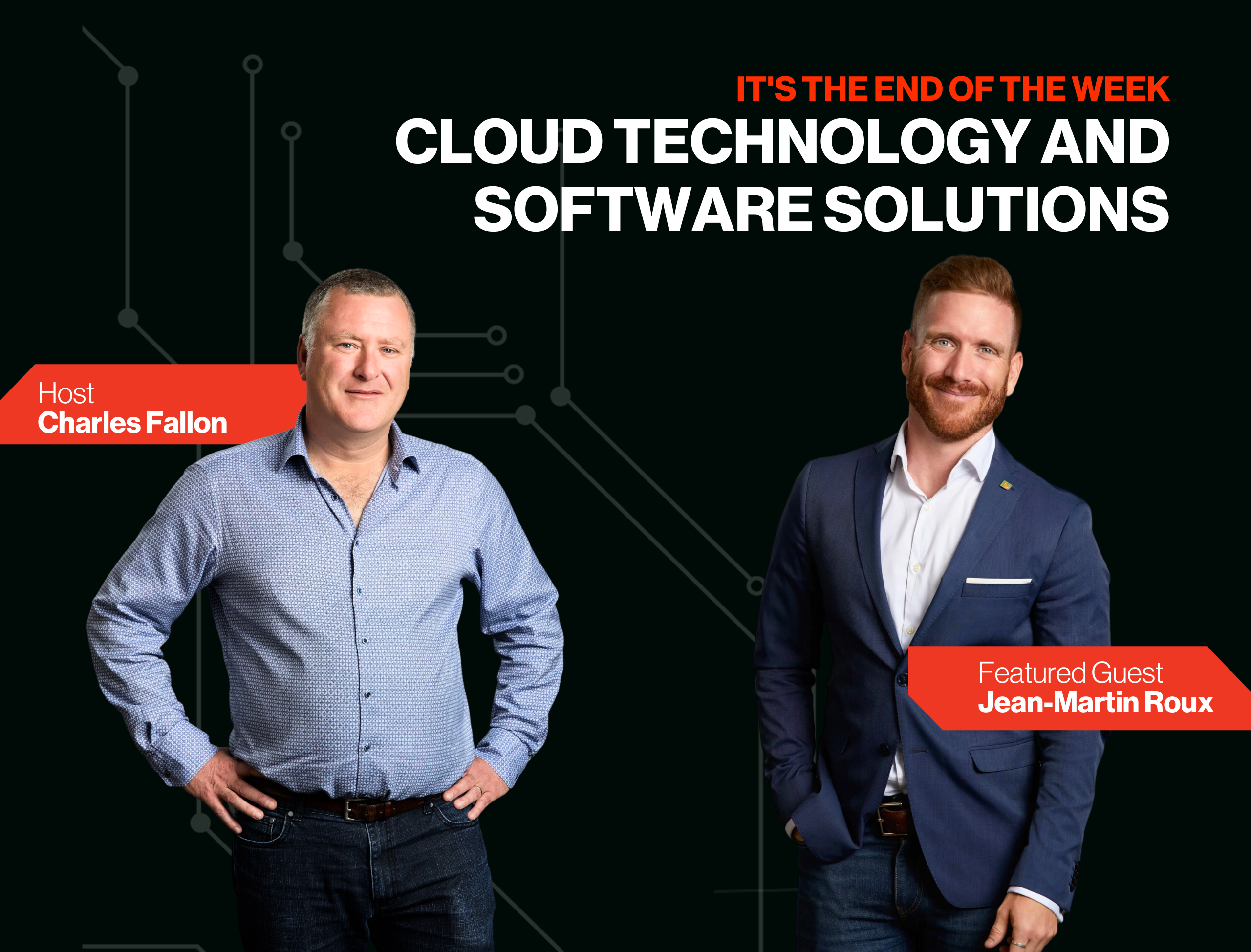
Is It Really up in the Air? Demystifying Cloud Technology and Software Solutions
Charles Fallon and Jean-Martin Roux work to demystify cloud infrastructure and technology as it rela...
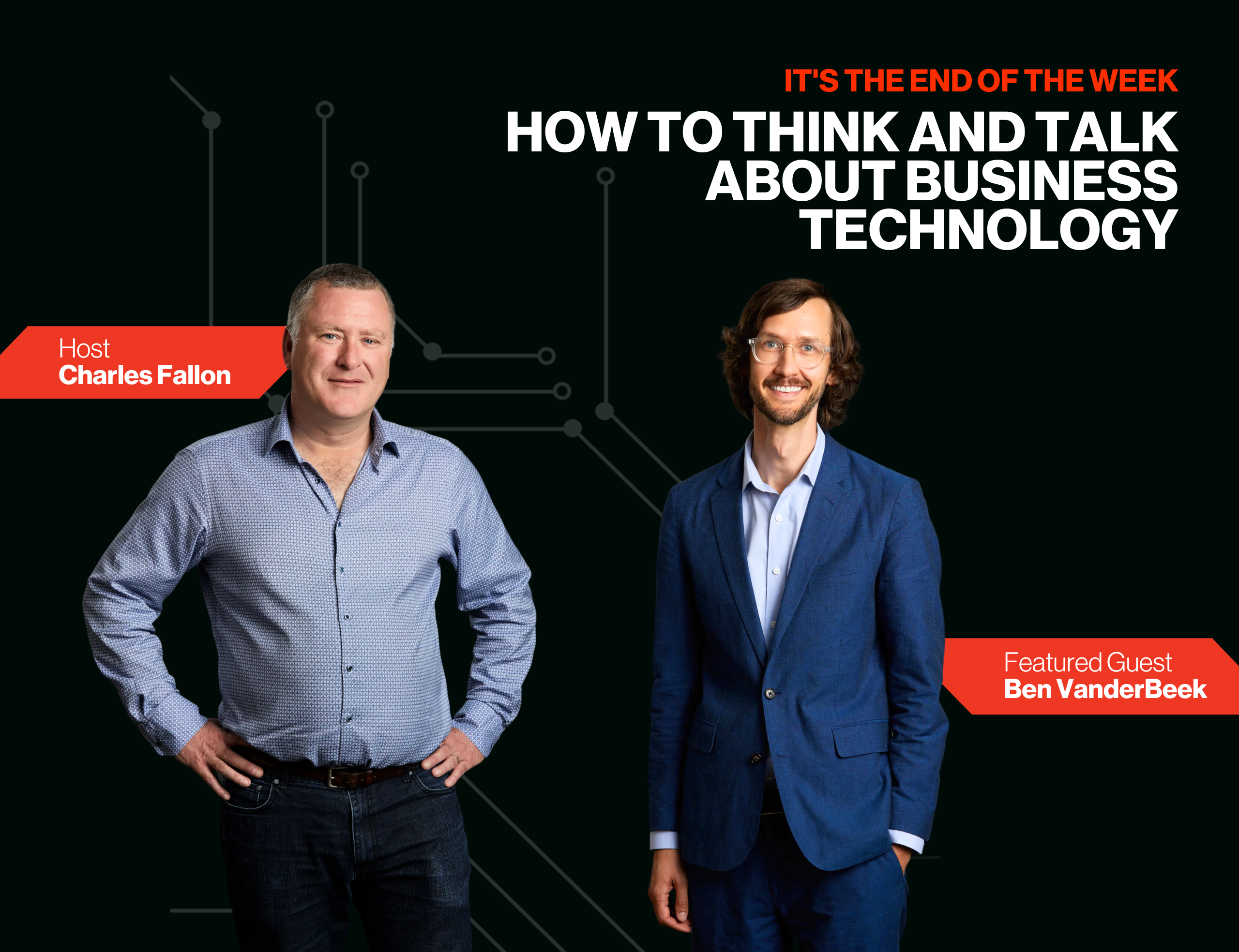
How to Think and Talk about Business Technology with Ben V.
LIDD founder, Charles Fallon, and guest speaker Ben VanderBeek, discuss how best to think and talk a...
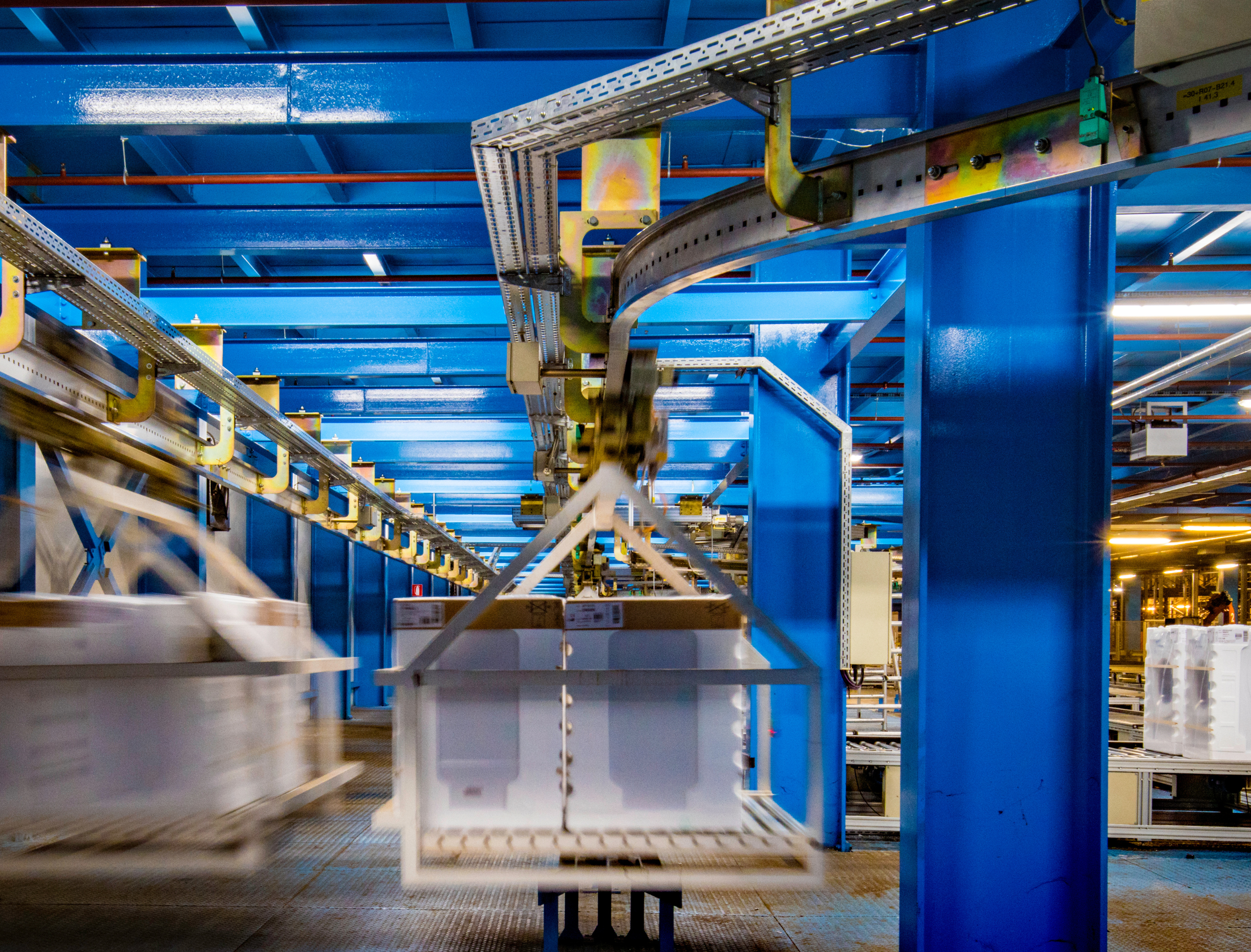
Warehouse Automation Projects: Insights from the “Unboxing Fulfillment Podcast”
Executing a successful warehouse automation project requires careful planning, data-driven decision-...
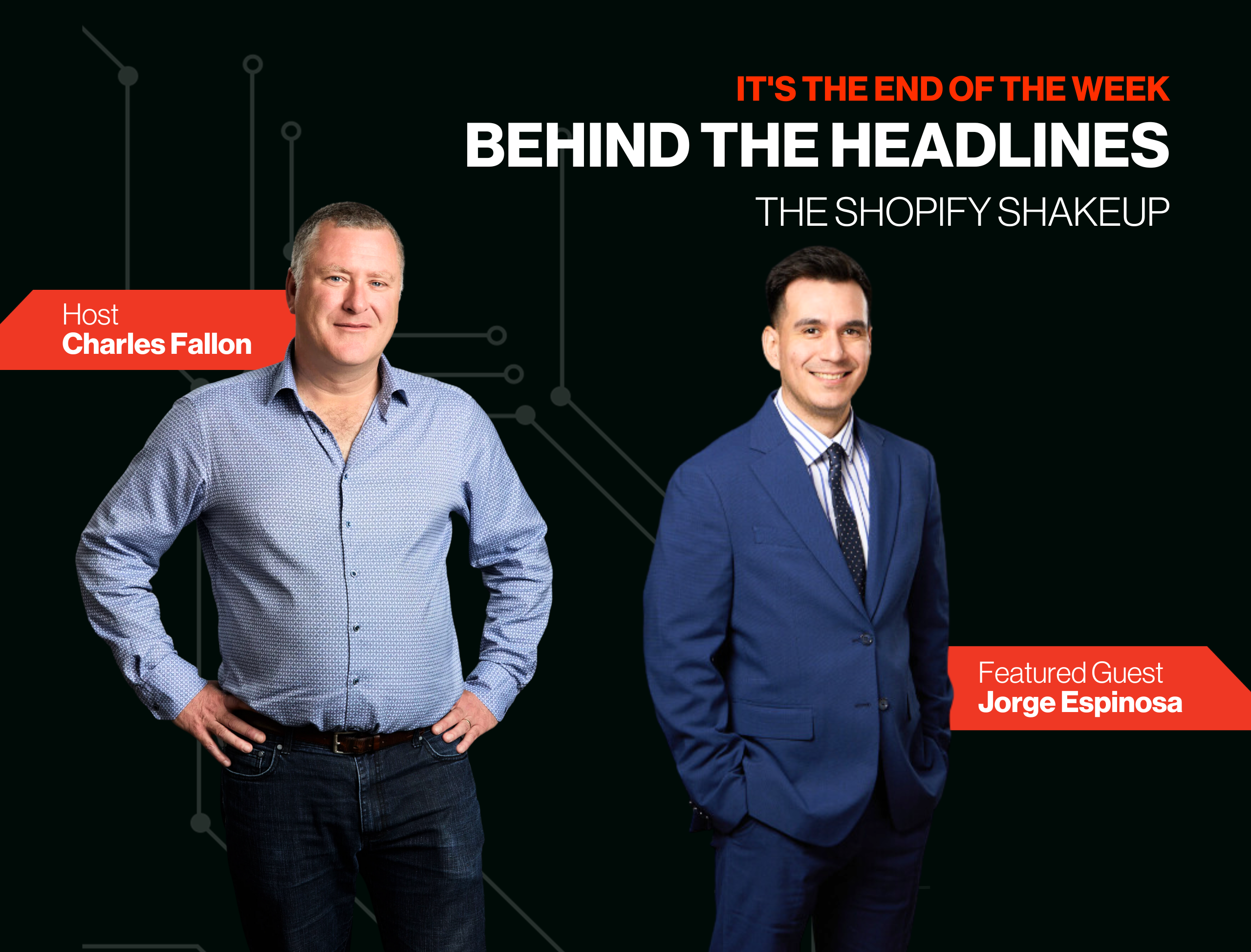
Shopify’s Workforce Cutbacks and Logistics Business Divestment
LIDD founder, Charles Fallon, and guest speaker Jorge Espinosa, discuss the rationale and implicatio...